Listen to this article ![]() |
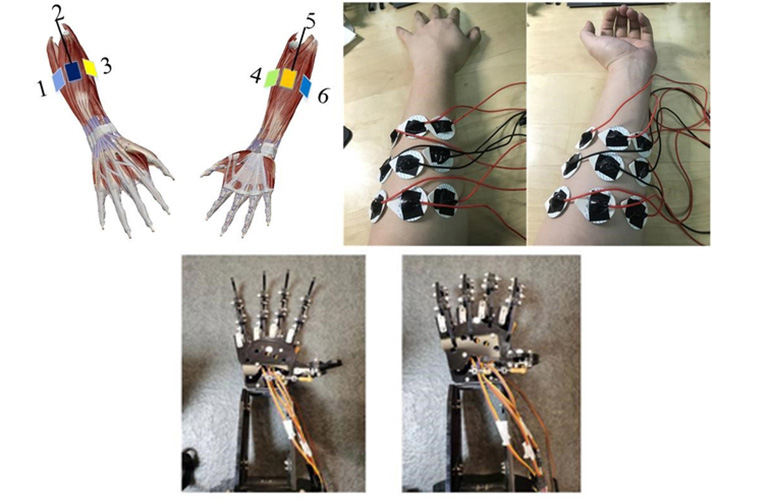
A schematic and physical diagram of the location of electrodes. Below, a prosthetic hand robot used by the team.
Researchers at Shenyang University of Technology and the University of Electro-Communications in Tokyo are trying to figure out how to make prosthetic hands respond to arm movements.
For the last decade, scientists have been trying to figure out how to use surface electromyography (EMG) signals to control prosthetic limbs. EMG signals are electrical signals that cause our muscles to contract. They can be recorded by inserting electrode needles into the muscle. Surface EMGs are recorded with electrodes placed on the skin above muscles.
Surface EMGs could be used to allows prosthetic limbs to respond faster, and move more naturally. However, interruptions, such as a shift in the electrodes, can make it hard for a device to recognize those signals. One way to overcome this is by doing surface EMG signal training. The training can be a long and at times difficult process for amputees.
So, many researchers have turned to machine learning. With machine learning, a prosthetic limb could learn the difference between muscle movements that indicate gestures, and movements of electrodes.
The authors of a study published in Cyborg and Bionic Systems developed a unique machine learning method that combined a convolutional neural network (CNN) and a long short-term memory (LSTM) artificial neural network. They landed on these two methods because of their complementing strengths.
A CNN does well at picking up on the spatial dimensions of surface EMG signals and understanding how they relate to hand gestures. It struggles with time. Gestures take place over time, but a CNN ignores time information in continuous muscle contractions. Typically, CNNs are used for image recognition.
LSTM is usually used for handwriting and speech recognition. This neural network is good at processing, classifying and making predictions based on sequences of data over time. They’re not very practical for prosthetics, however, because the size of the computational model would be too costly.
The research team created a hybrid model, combining the spacial awareness of CNN and temporal awareness of LSTM. In the end, they had reduced the size of the deep learning model, and still maintained high accuracy and a strong resistance to interference.
The system was tested on ten non-amputee subjects with a series of 16 different hand gestures. The system had a recognition accuracy of over 80%. It did well with most gestures, like holding a phone or pen, but struggled with pinching using it’s middle and index fingers. Overall, according to the team, the results outpaced traditional learning methods.
The end goal for the researchers is to develop a flexible and reliable prosthetic hand. Their next steps are to further improve accuracy of the system, and figure out why it struggled with the pinching gestures.
Credit: Source link
Comments are closed.