DeepMind Researchers Develop Ithaca: A Deep Neural Network For Restoring and Attributing Ancient Texts

Epigraphy is the study of ancient texts—inscriptions—written by individuals, organizations, and institutions in the ancient world on durable materials (stone, pottery, metal). Thousands of inscriptions have survived to the present day, but many have been destroyed through time, and their texts are now incomplete. Inscriptions can also be transported or trafficked far from their initial place, and radiocarbon dating isn’t possible because most inscribed supports are inorganic.
The missing text must then be reconstructed, a process known as text restoration, and the original location and date of writing must be determined, jobs known as geographical attribution and chronological attribution, respectively. These three objectives are critical in establishing an inscription’s place in history as well as in the lives of those who wrote and read it. These tasks, however, are not simple, and standard epigraphic procedures include highly complex, time-consuming, and specialized workflows.
Epigraphers use enormous libraries of information to uncover textual and contextual analogies while reconstructing damaged inscriptions. These archives generally contain a researcher’s parallel mnemonic repertoire and, more recently, digital corpora for performing string matching’ searches. Differences in the search query, on the other hand, can remove or confuse pertinent results, and estimating the true probability distribution of possible restorations is nearly impossible.
Attributing an inscription is also difficult—if it has been moved or if crucial internal dating factors are lacking, historians must devise new criteria to assign the writing’s location and date (such as letterforms, dialects). Inevitably, there is a significant level of generalization involved (chronological attribution intervals can be very long).
DeepMind researchers used cutting-edge machine learning research to overcome the limitations of current epigraphic methods in a new publication. Deep neural networks, which are based on biological neural networks, can find and exploit complicated statistical patterns in large amounts of data. Recent advances in computing capacity have enabled these models to take on increasingly complex issues in a variety of domains, including the study of ancient languages.
Ithaca is a deep neural network architecture trained to execute textual restoration, geographical attribution, and chronological attribution tasks all at the same time, according to the team. Between the seventh century BC to the fifth century AD, Ithaca was trained on inscriptions written in the ancient Greek language and across the ancient Mediterranean globe and was called after the Greek island that eluded the hero Odysseus’ return.
This decision was made for two reasons. The availability of digital corpora for ancient Greek, a vital resource for training machine learning models, and first, the variety of contents and context of the Greek epigraphic record makes it a good challenge for language processing.
The team built a pipeline to retrieve the unprocessed Packard Humanities Institute (PHI) dataset, which contains the transcribed texts of 178,551 inscriptions, in order to train Ithaca. This process involved making the text machine-actionable, standardizing epigraphic notations, eliminating noise, and efficiently dealing with all abnormalities. Each PHI inscription has a unique numerical ID and is labeled with details about the writing location and time.
PHI includes 84 ancient regions, but the chronological information is recorded in a variety of formats, ranging from historical eras to precise year intervals, written in multiple languages, lacking in conventional notation, and frequently employing ambiguous wording. The final dataset I.PHI, which contains 78,608 inscriptions and was created using an expanded ruleset to analyze and filter the data (Methods), is the largest multitask dataset of machine-actionable epigraphical text that the team is aware of.
Ithaca’s design was carefully tuned to each of the three epigraphic activities, processing long-term context information and delivering interpretable outputs to maximize the possibility for human-machine collaboration. To begin, by encoding the inputs as words, more contextual information is collected; yet, sections of words may have been lost through the years. To overcome this problem, researchers combine character and word representations in the input text, using the special symbol ‘[unk]’ to represent broken, missing, or unknown words.
Ithaca’s torso is built on the transformer, a neural network design that uses an attention mechanism to weigh the importance of different sections of the input (such as characters) on the model’s decision-making process, allowing for large-scale processing. By concatenating the input character and word representations with their sequential positional information, the attention mechanism is aware of the position of each part of the input text. The body of Ithaca is made up of stacked transformer blocks, each of which outputs a sequence of processed representations with a length equal to the number of input characters, and each block’s output becomes the input of the next.
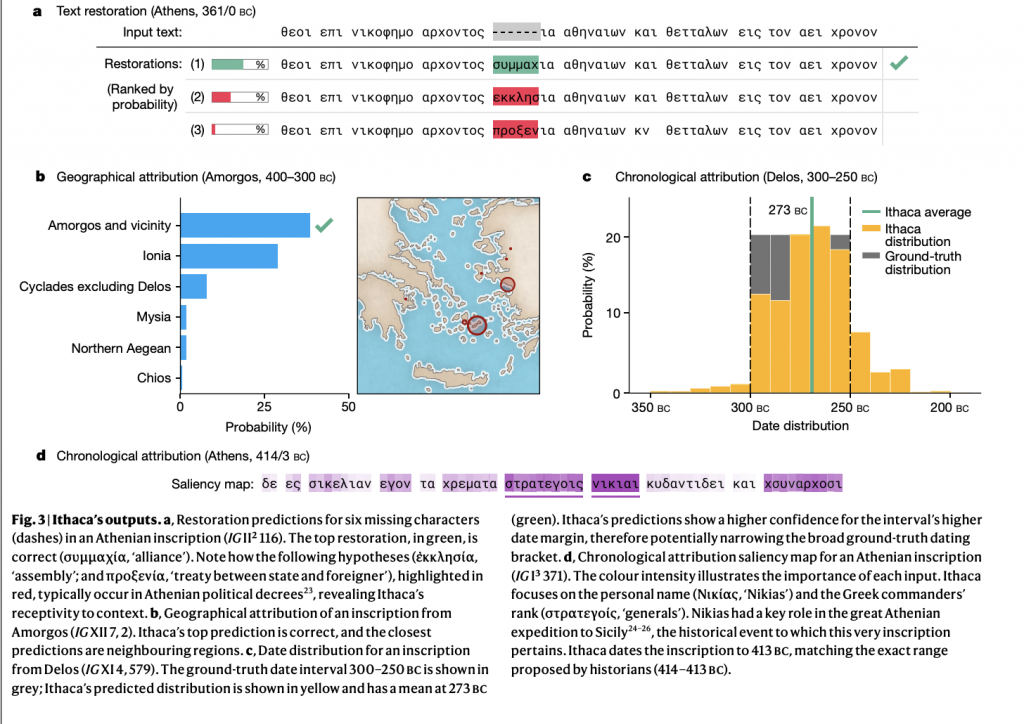
Ithaca consistently outperforms rival approaches for restoration, obtaining a 26.3 percent CER and 61.8 percent top 1 accuracy. In particular, when compared to human experts, the model obtains 2.2 times lower (that is, better) CER, while Ithaca’s top 20 predictions perform 1.5 times better than Pythia, with an accuracy of 78.3 percent. Human experts attain an 18.3 percent CER and 71.7 percent top 1 accuracy when teaming historians with Ithaca (ancient historian and Ithaca), exhibiting a significant 3.2 times and 2.8 improvement times over their original CER and top 1 scores. Ithaca has 70.8 percent top 1 and 82.1 percent top 3 prediction accuracy when it comes to attribution to areas.
The experimental evaluation effectively reveals Ithaca’s influence on inscription research and, as a result, their historical significance. To begin, Ithaca can uncover epigraphic patterns on an unprecedented scale and in unprecedented depth, utilizing large amounts of epigraphic data to achieve high performance in all three epigraphic tasks. Furthermore, while Ithaca may have surpassed historians in the first baseline when a historian’s own (contextual) knowledge was combined with Ithaca’s helpful input, the model’s performance was improved even more.
Conclusion
Ithaca is the first of its sort in terms of epigraphic restoration and attribution. It may aid the restoration and attribution of newly discovered or uncertain inscriptions, transforming their value as historical sources and assisting historians in gaining a more holistic understanding of the distribution and nature of epigraphic habits across the ancient world by significantly improving the accuracy and speed of the epigrapher’s pipeline. To do this, the interdisciplinary team developed an open-source, publicly accessible interface that allows historians to utilize Ithaca for their own study while also allowing for future improvement.
Paper: https://www.nature.com/articles/s41586-022-04448-z.pdf
Reference: https://deepmind.com/blog/article/Predicting-the-past-with-Ithaca
Suggested
Credit: Source link
Comments are closed.