Listen to this article ![]() |
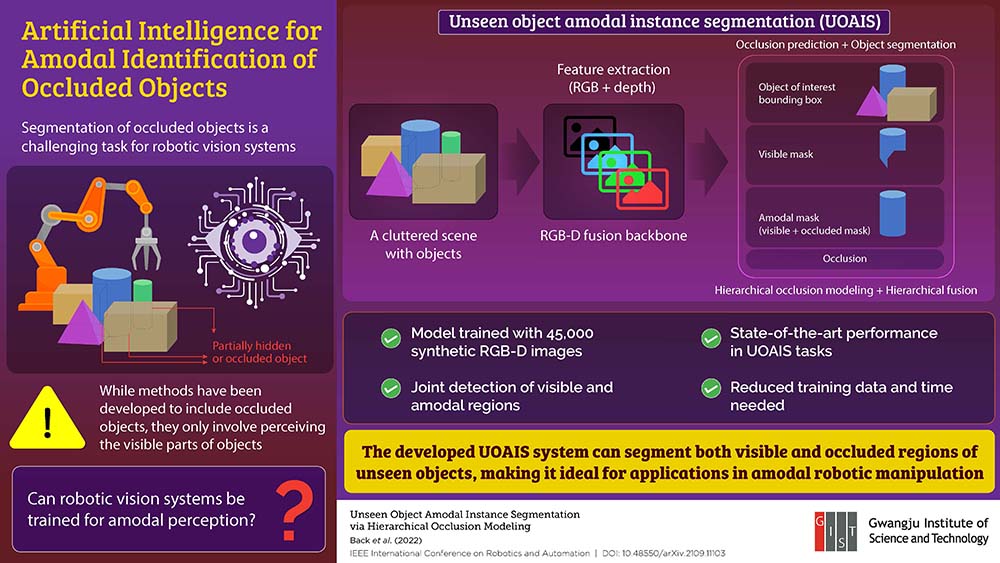
| Source: Gwangju Institute of Science and Technology
A research team at the Gwangju Institute of Science and Technology (GIST) in Korea created a method to allow artificial intelligence (AI) vision systems to better identify objects that are cluttered together and may not be entirely visible.
Kyoobin Lee, an associate professor at GIST, and Seunghyeok Back, a Ph.D. student, and their research team at the university, set out to create an AI system that could identify and sort objects in cluttered scenes. The team quickly found that robotic vision systems require large datasets of objects to be able to identify objects are aren’t fully visible.
“We expect a robot to recognize and manipulate objects they have not encountered before or been trained to recognize,” Back said. “In reality, however, we need to manually collect and label data one by one as the generalizability of deep neural networks depends highly on the quality and quantity of the training dataset.”
Typically, when AI is presented with a scene of many cluttered objects, the system will try to identify each item based only on the parts of it that are visible. Lee and Back decided to take a different approach, and instead taught the AI to recognize the geometry of each object, so that it can infer what parts of the object it can’t see.
Teaching the AI to do this required a much smaller dataset of 45,000 photo realistic synthetic images containing depth information. When the AI is presented with a scene, it begins to understand it by picking out an object of interest and then segmented the object into a “visible mask” and an “amodal mask”.
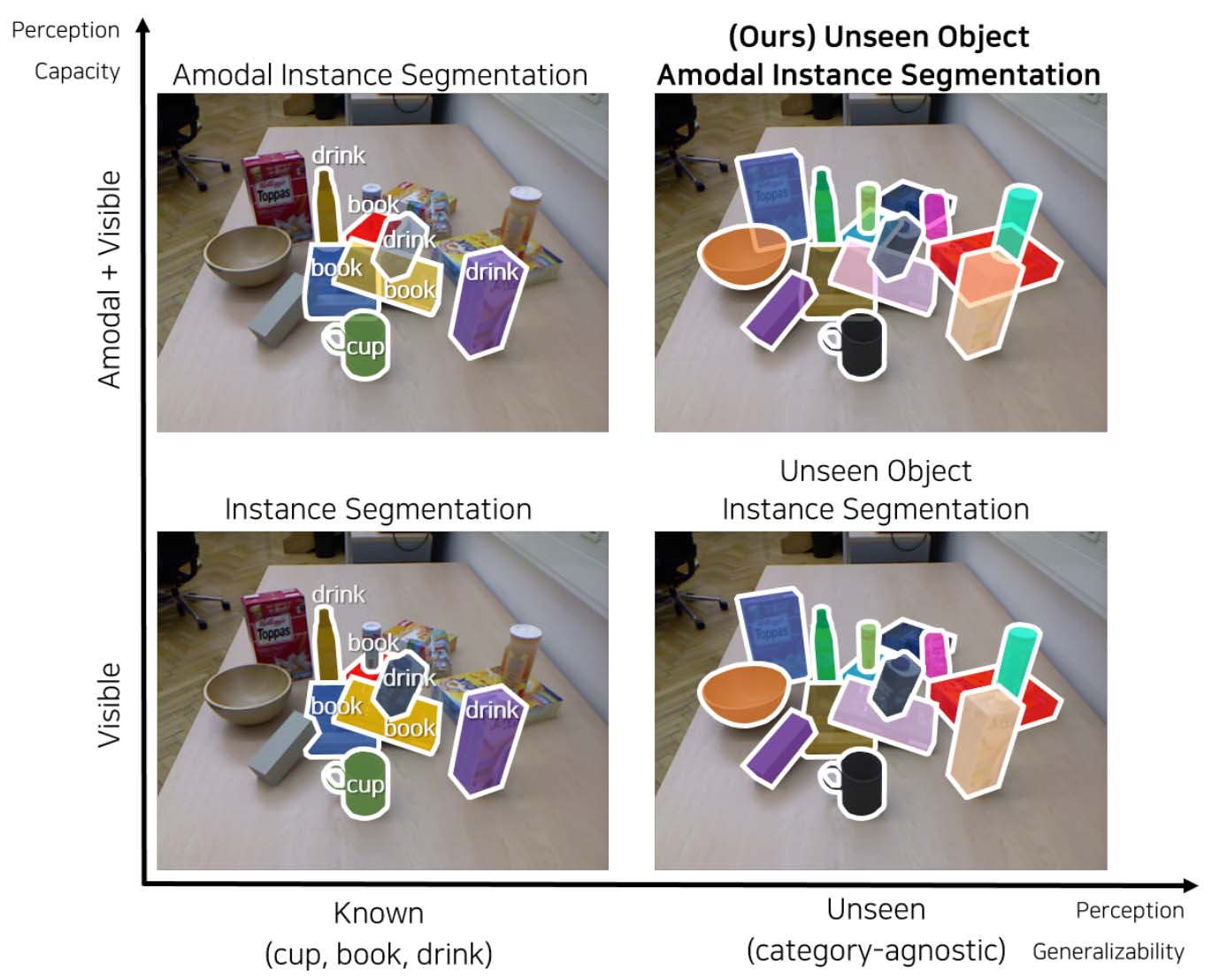
Comparing existing methods and the researcher’s method of identifying partially obscured objects. | Source: Gwangju Institute of Science and Technology
After segmenting the scene, the AI uses a hierarchical occlusion modeling (HOM) scheme. The scheme ranks combinations of features that could be obscured by how likely they may be present. The team tested its HOM scheme against three benchmarks and found that it achieved state-of-the-art performance.
“Previous methods are limited to either detecting only specific types of objects or detecting only the visible regions without explicitly reasoning over occluded areas,” Back said. “By contrast, our method can infer the hidden regions of occluded objects like a human vision system. This enables a reduction in data collection efforts while improving performance in a complex environment.”
The research team’s results were accepted at the 2022 IEEE International Conference on Robotics and Automation.
Credit: Source link
Comments are closed.