How lenders can leverage technology to mitigate duplicate trade fraud.
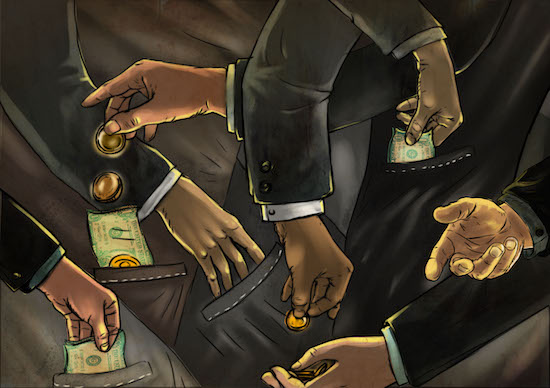
How lenders can leverage technology to mitigate duplicate trade fraud
Trade finance fraud is not a new financial crime, but it has been resurfacing in headlines increasingly in recent years. This may be partly due to the post-Covid demand on the supply chain. However, others believe that it has more to do with the number of high-profile banks moving away from the funding trade because of growth in fraud that has been plaguing this line of business lending, which historically offered banks a significant return on their investment.
Before we can explore how technology can assist in providing a defensive, preventative posture and how standardisation can improve the outlook for the future of trade financing, we need to understand what has changed and examine the different aspects of trade fraud.
Trade financing fraud costs are astronomical
In July 2020, a livemint.com headline read: “After $9 billion credit hit, banks seek trade finance overhaul.” This scam not only left more than 23 banks on the hook for $3.5 billion dollars, but it also put the perpetrator, Hin Leong Trading Pte – one of Singapore’s biggest oil traders at the time – on the road to collapse.
This story marks the day the banking world was jolted into taking notice of the anti-fraud gaps within trade financing – gaps that still exist today and are pushing FIs to adopt better practices and new technologies. Over the past few months, we have made it our mission to understand firsthand from financial institutions why this gap persists. Some have cited that it’s easier to withdraw altogether from trade financing as a business model than to take on the cost of trade financing fraud or even the cost of fraud prevention.
Let’s examine one type of trade financing fraud known as duplicate trade financing. Traditional fraud is the intentional deception to secure unfair and unlawful gain, but duplicate trade financing fraud is the act of premeditatively seeking financing from multiple sources to fund the same invoice, purchase order, accounts receivable etc. While there are many reasons why duplicate trade financing fraud plagues reputable and noteworthy financial institutions, most include the challenges of implementing a sufficient defensive posture – due to antiquated processes and behaviors, lack of standardisation, and limitations on how technology can help prevent and mitigate risk.
Nefarious actors leverage these communication breakdowns to exploit financial institutions. Most lenders have their own proprietary data systems and do not share customer data across borders or with other lenders – easily enabling bad actors to secure duplicate financing from multiple financial institutions for the same order. This was precisely the case with Hin Leong Trading, who shopped around financing for a $76.5 million dollar fuel purchase to multiple banks including HSBC and Credit Agricole, who eventually funded the fuel purchase, only to fall victim to a duplicate financing scam, along with 21 other financial institutions. The banks that were hardest hit each lost at least $400-$600 million, just from this one organisation.
As technology continues to advance and new regulations are being introduced that empower organisations to do more with sensitive data – financial institutions are still falling behind on strategically leaning on modern IT to close the gap on trade financing fraud.
The impact of privacy in trade financing
There are many challenges that arise for financial institutions in developing a strategy to detect and prevent trade financing fraud. These challenges are not limited to, but include:
Privacy regulation differs by country and is one of the most critical aspects of carrying out a collaborative data sharing strategy. Cross-institution and cross-border collaboration can only occur while maintaining regulatory compliance, and technology must account for this.
Competitive concerns or protecting one’s intellectual property (IP) is also a critical factor when enabling collaboration. Financial institutions have their own competitive landscape; they want to ensure that customer retention – and, ultimately, capitalisation – is not impacted by giving too much of their data away.
Financial regulations that impact the trade financing process are measures which aim to stabilise and protect the financial system, as well as protect consumers. These regulations can cover financial crimes, such as money laundering and fraud, and can also address banking secrecy rules which (in some jurisdictions) forbid financial institutions from revealing certain business relationships. These regulations present their own set of obstacles in finding the balance between protecting data privacy and regulatory compliance.
Cross-bank and cross-border data sharing refers to the ability or agreement to share sensitive data with other financial institutions either in a country, region or across borders to conduct discovery on potential bad actors or fraudsters who are shopping their financing options around. As was in the case that we referred to earlier, that unwittingly propagated into a series of duplicate trade finance scandals that led to more than $9 billion in losses for global lenders.
Opportunities are abundant to earn revenue by establishing better safeguards and leveraging technology to implement an overhaul of trade finance transactions. Nevertheless, several obstacles remain to the adoption of modern IT, as it requires knowledge, expert guidance, and the ability to leverage technological investments over time – with the understanding that an investment today should also support the goals of tomorrow.
Three key areas to consider when driving a strategic shift in technology that will enable organisations to collaborate in combating fraud threats while ensuring privacy is preserved are: encryption types, approaches, and technologies; data collaboration platform adoption vs adoption of a single privacy enhancing technology; and the impact of data modelling and AI.
Privacy enhancing technologies (PETs) vs platform, the PETs landscape consists of several different types of privacy enhancing technologies that are used to preserve, encrypt, and contend with sensitive data while ensuring shareability.
The most common PETs used by financials vary based on need:
- Federated learning, a method of statistical analysis or model training on decentralised data sets. With federated learning, the algorithm “travels” between data sets and makes the model “smarter” every time the data undergoes new analysis.
- Differential privacy, a data aggregation method which adds randomised “noise” to the data; data cannot be reverse engineered to understand the original inputs.
- Homomorphic encryption and/or encryption in use, wherein sensitive data is always secured, regardless of where it is in the data lifecycle or where it is being stored, yet still being able to analyse it.
- Synthetic data, creating data artificially to replace/represent real-world sensitive data.
- Trusted execution environments, a hardware-based, physically isolated execution environment (usually a secure area of a main processor) that guarantees code and data loaded inside to be protected.
This is in addition to, or in place of, other measures that financial institutions have started to leverage to address the trade financing fraud challenge:
- Hashing, or transforming the data/string of characters into a different value, using a formula (“hash”). The problem is that these are best for exact matches, making it easy for criminals to circumnavigate these checks. A fraudster trying to hide duplicate financing can easily use different purchase order numbers, for example. Banks need technology that is fit for purpose and able to detect the more complex tactics criminals deploy, including uncovering suspicious patterns across institutions.
- Blockchain, i.e. storing data in a closed network – but even in a private blockchain, any participant can see the data being shared, leaving it vulnerable to be shared outside a private network and potentially falling afoul of privacy laws, as well as competitive concerns.
The privacy preserving data collaboration experts at Duality recommend that you identify the situations where data privacy is crucial, determine how data needs to be shared, and understand what exactly needs to be protected.
Then you can select which PET or combination of PETs will best suit your use case. No one PET suits all situations, and it’s critical for financial services organisations to conduct a review of whether PETs are already being implemented, what PETs exist across the data sources and organisations they are looking to collaborate with, and from there establish the right combination of PET(s) to achieve the goals.
This scenario is very similar to the more traditional security threats of the past: it’s not enough to intercept a single point of vulnerability with a single product; rather, one must think more broadly about infrastructure and the many different aspects of fraud prevention.
Once you have defined your PET landscape and the criteria list required in a solution that will support your data collaboration objectives, it’s time to weigh the benefits of adding data modelling and AI to your strategy.
Data modelling is the process used to define and analyse data requirements needed to support the business processes within the scope of corresponding information systems in organisations. Therefore, the process of data modelling involves leveraging professionals with deep roots in data science and working closely with business stakeholders as well as potential users of the information system.
And while data modeling falls into three distinct categories or types – conceptual, logical, and physical – we recommend that financial institutions focus on the techniques and methodologies that enable data sharing to be standardised with predictable and actionable outcomes.
Working closely with a trusted advisor that helps establish models to support privacy preserving data collaboration enables financial institutions to establish a dependable, preemptive strategy to tackle fraud and mitigate risk.
Erick Brethenoux, a Gartner analyst, published an article in March, “What is artificial intelligence? Ignore the hype; here’s where to start”, where he challenges data and analytics leaders to “demystify AI” by removing the technology jargon and focusing the conversation on solving real business problems with achievable use cases.
First, Brethenoux speaks my language, which may sound interesting knowing that I’m responsible for the marketing practice at Duality – and we all know that marketing is synonymous with trendy jargon. However, supporting the goals of technology today must be less about “marketing hyperbole” (how Brethenoux refers to it) and more about actually solving critical problems. When it comes to fraud, we all pay in the end as it has a global impact. Therefore, when it comes to preventing it, we all win.
So how does AI impact data modelling, or more specifically financial fraud? From the fraud perspective this would fall into what Gartner refers to in its AI Techniques Framework as predictive modelling, a method of predicting future outcomes by using data modelling. Financial institutions must first embrace data collaboration, then apply AI to their data modelling strategy, advancing their analysis and logic-based techniques – including machine learning (ML) to interpret events, support and automate decisions, and drive actionable outcomes.
Identifying the data sources, data types, geographic footprint, PETs, and goals are all part of the discovery checklist that any financial institution which is looking to solve a fraud problem holistically must adhere to – and what our organisation helps financials solve.
Our economy is exceedingly global, our dependency on all things digital continues to grow, and fraud is easier to pull off if we don’t solicit and unleash the power of our data.
Think global, act local
Howard Zinn said it best, “We don’t have to engage in grand, heroic actions to participate in the process of change. Small acts, when multiplied by millions of people, can transform the world.”
Financial institutions can take small steps first, such as kicking off an intra-banking (cross-border or cross-team) collaboration project, then shift to working with other financial institutions to leverage each other’s data in a regulatory compliant manner.
The adoption of modern IT, data science and cryptography enable a more advanced approach to data sharing and collaboration which ensures that privacy is preserved, complex data sharing and multisource data is possible to use – and, ultimately, that financials have a proven strategy that they can lean on to mitigate risks, account for bad characters and behaviors, and unleash the value of the data they are already collecting.
Duality is the leader in privacy enhanced secure data collaboration, empowering organisations worldwide to maximise the value of their data without compromising on privacy or regulatory compliance.
Learn more about trade finance with Duality and how you can help drive change and contribute to the evolution of financial standards for privacy preserving data collaboration.
By Marcella Arthur, VP, worldwide marketing, Duality
About the author
Marcella Arthur is the vice president of worldwide marketing at Duality and is responsible for leading the marketing strategy and its integration to the business’ go-to-market.
With over two decades of technology experience, Arthur is passionate about the interaction between security and data and fervently believes that enterprises need to maximise the value of their data.
Credit: Source link
Comments are closed.