This week two new, but contrasting AI-driven graphics algorithms are offering novel ways for end users to make highly granular and effective changes to objects in photos.
The first is Imagic, from Google Research, in association with Israel’s Institute of Technology and Weizmann Institute of Science. Imagic offers text-conditioned, fine-grained editing of objects via the fine-tuning of diffusion models.

Change what you like, and leave the rest – Imagic promises granular editing of only the parts that you want to be changed. Source: https://arxiv.org/pdf/2210.09276.pdf
Anyone who has ever tried to change just one element in a Stable Diffusion re-render will know only too well that for every successful edit, the system will change five things that you liked just the way they were. It’s a shortcoming that currently has many of the most talented SD enthusiasts constantly shuffling between Stable Diffusion and Photoshop, to fix this kind of ‘collateral damage’. From this standpoint alone, Imagic’s achievements seem notable.
At the time of writing, Imagic as yet lacks even a promotional video, and, given Google’s circumspect attitude to releasing unfettered image synthesis tools, it’s uncertain to what extent, if any, we’ll get a chance to test the system.
The second offering is Runway ML’s rather more accessible Erase and Replace facility, a new feature in the ‘AI Magic Tools’ section of its exclusively online suite of machine learning-based visual effects utilities.
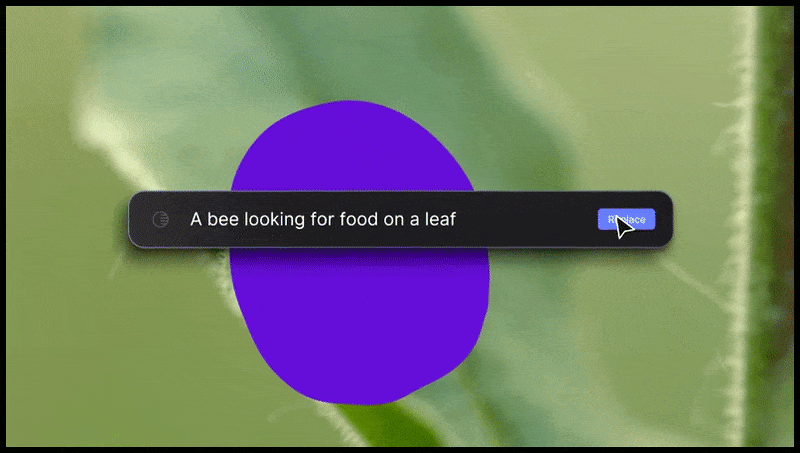
Runway ML’s Erase and Replace feature, already seen in a preview for a text-to-video editing system. Source: https://www.youtube.com/watch?v=41Qb58ZPO60
Let’s take a look at Runway’s outing first.
Erase and Replace
Like Imagic, Erase and Replace deals exclusively with still images, though Runway has previewed the same functionality in a text-to-video editing solution that’s not yet released:
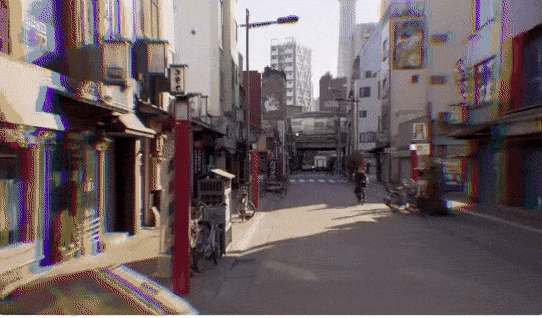
Though anyone can test out the new Erase and Replace on images, the video version is not yet publicly available. Source: https://twitter.com/runwayml/status/1568220303808991232
Though Runway ML has not released details of the technologies behind Erase and Replace, the speed at which you can substitute a house plant with a reasonably convincing bust of Ronald Reagan suggests that a diffusion model such as Stable Diffusion (or, far less likely, a licensed-out DALL-E 2) is the engine that’s reinventing the object of your choice in Erase and Replace.
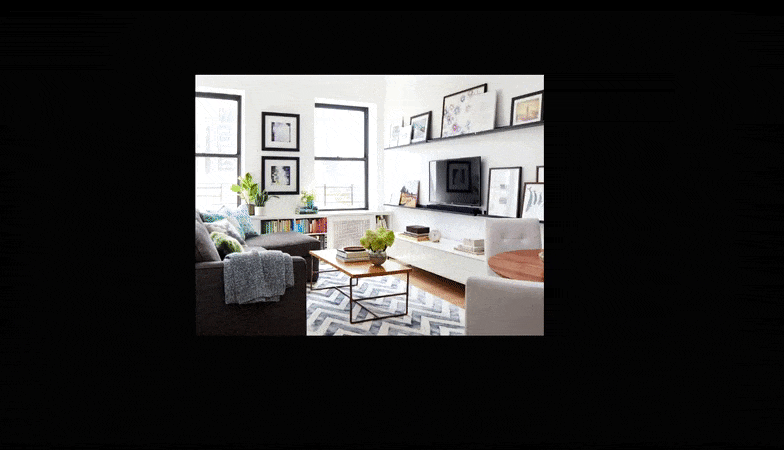
Replacing a house plant with a bust of The Gipper isn’t quite as fast as this, but it’s pretty fast. Source: https://app.runwayml.com/
The system has some DALL-E 2 type restrictions – images or text that flag the Erase and Replace filters will trigger a warning about possible account suspension in the event of further infractions – practically a boilerplate clone of OpenAI’s ongoing policies for DALL-E 2 .
Many of the results lack the typical rough edges of Stable Diffusion. Runway ML are investors and research partners in SD, and it’s possible that they have trained a proprietary model that’s superior to the open source 1.4 checkpoint weights that the rest of us are currently wrestling with (as many other development groups, hobbyist and professional alike, are currently training or fine-tuning Stable Diffusion models).
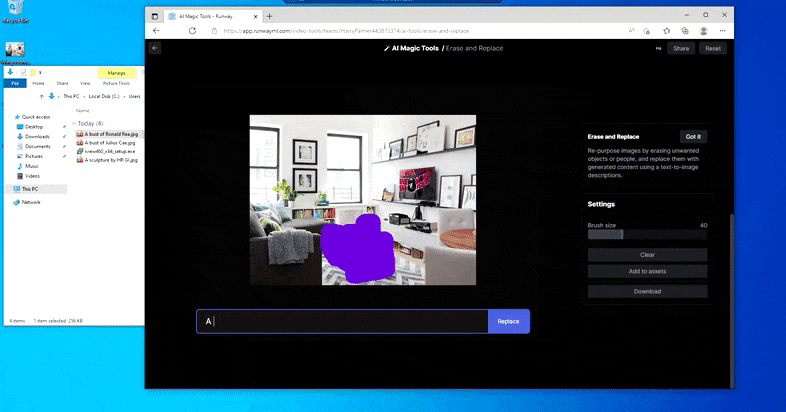
Substituting a domestic table for a ‘table made of ice’ in Runway ML’s Erase and Replace.
As with Imagic (see below), Erase and Replace is ‘object-oriented’, as it were – you can’t just erase an ’empty’ part of the picture and inpaint it with the result of your text prompt; in that scenario, the system will simply trace the nearest apparent object along the mask’s line-of-sight (such as a wall, or a television), and apply the transformation there.
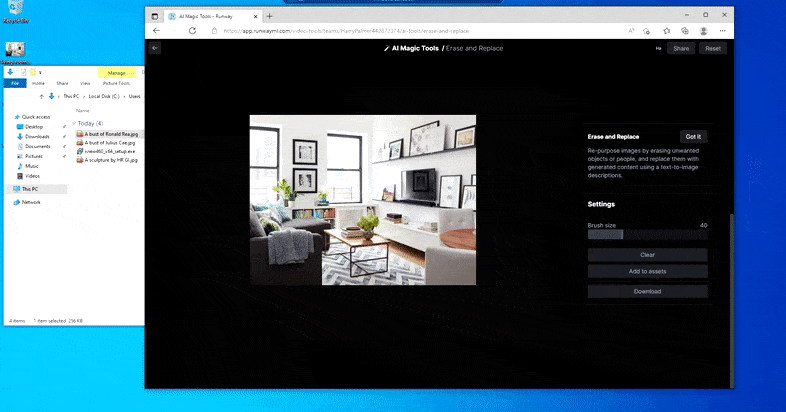
As the name indicates, you can’t inject objects into empty space in Erase and Replace. Here, an effort to summon up the most famous of the Sith lords results in a strange Vader-related mural on the TV, roughly where the ‘replace’ area was drawn.
It is difficult to tell if Erase and Replace is being evasive in regard to the use of copyrighted images (which are still largely obstructed, albeit with varying success, in DALL-E 2), or if the model being used in the backend rendering engine is just not optimized for that kind of thing.

The slightly NSFW ‘Mural of Nicole Kidman’ indicates that the (presumably) diffusion-based model at hand lacks DALL-E 2’s former systematic rejection of rendering realistic faces or racy content, while the results for attempts to evince copyrighted works range from the ambiguous (‘xenomorph’) to the absurd (‘the iron throne’). Inset bottom right, the source picture.
It would be interesting to know what methods Erase and Replace is using to isolate the objects that it is capable of replacing. Presumably the image is being run through some derivation of CLIP, with the discrete items individuated by object recognition and subsequent semantic segmentation. None of these operations work anywhere near as well in a common-or-garden installation of Stable Diffusion.
But nothing’s perfect – sometimes the system seems to erase and not replace, even when (as we have seen in the image above), the underlying rendering mechanism definitely knows what a text prompt means. In this case, it proves impossible to turn a coffee table into a xenomorph – rather, the table just disappears.
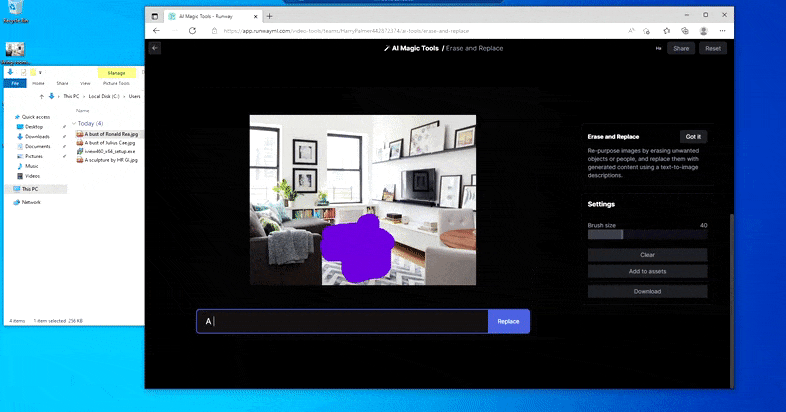
A scarier iteration of ‘Where’s Waldo’, as Erase and Replace fails to produce an alien.
Erase and Replace appears to be an effective object substitution system, with excellent inpainting. However, it can’t edit existing perceived objects, but only replace them. To actually alter existing image content without compromising ambient material is arguably a far harder task, bound up with the computer vision research sector’s long struggle towards disentanglement in the various latent spaces of the popular frameworks.
Imagic
It’s a task that Imagic addresses. The new paper offers numerous examples of edits that successfully amend individual facets of a photo while leaving the rest of the image untouched.

In Imagic, the amended images do not suffer from the characteristic stretching, distortion and ‘occlusion guessing’ characteristic of deepfake puppetry, which utilizes limited priors derived from a single image.
The system employs a three-stage process – text embedding optimization; model fine-tuning; and, finally, the generation of the amended image.

Imagic encodes the target text prompt to retrieve the initial text embedding, and then optimizes the result to obtain the input image. After that, the generative model is fine-tuned to the source image, adding a range of parameters, before being subjected to the requested interpolation.
Unsurprisingly, the framework is based on Google’s Imagen text-to-video architecture, though the researchers state that the system’s principles are broadly applicable to latent diffusion models.
Imagen uses a three-tier architecture, rather than the seven-tier array used for the company’s more recent text-to-video iteration of the software. The three distinct modules comprise a generative diffusion model operating at 64x64px resolution; a super-resolution model that upscales this output to 256x256px; and an additional super-resolution model to take output all the way up to 1024×1024 resolution.
Imagic intervenes at the earliest stage of this process, optimizing the requested text embedding at the 64px stage on an Adam optimizer at a static learning rate of 0.0001.

A master-class in disentanglement: those end-users that have attempted to change something as simple as the color of a rendered object in a diffusion, GAN or NeRF model will know how significant it is that Imagic can perform such transformations without ‘tearing apart’ the consistency of the rest of the image.
Fine tuning then takes place on Imagen’s base model, for 1500 steps per input image, conditioned on the revised embedding. At the same time, the secondary 64px>256px layer is optimized in parallel on the conditioned image. The researchers note that a similar optimization for the final 256px>1024px layer has ‘little to no effect’ on the final results, and therefore have not implemented this.
The paper states that the optimization process takes approximately eight minutes for each image on twin TPUV4 chips. The final render takes place in core Imagen under the DDIM sampling scheme.
In common with similar fine-tuning processes for Google’s DreamBooth, the resulting embeddings can additionally be used to power stylization, as well as photorealistic edits that contain information drawn from the wider underlying database powering Imagen (since, as the first column below shows, the source images do not have any of the necessary content to effect these transformations).

Flexible photoreal movement and edits can be elicited via Imagic, while the derived and disentangled codes obtained in the process can as easily be used for stylized output.
The researchers compared Imagic to prior works SDEdit, a GAN-based approach from 2021, a collaboration between Stanford University and Carnegie Mellon University; and Text2Live, a collaboration, from April 2022, between the Weizmann Institute of Science and NVIDIA.

A visual comparison between Imagic, SDEdit and Text2Live.
It’s clear that the former approaches are struggling, but in the bottom row, which involves interjecting a massive change of pose, the incumbents fail completely to refigure the source material, compared to a notable success from Imagic.
Imagic’s resource requirements and training time per image, while short by the standards of such pursuits, makes it an unlikely inclusion in a local image editing application on personal computers – and it isn’t clear to what extent the process of fine-tuning could be scaled down to consumer levels.
As it stands, Imagic is an impressive offering that’s more suited to APIs – an environment Google Research, chary of criticism in regard to facilitating deepfaking, may in any case be most comfortable with.
First published 18th October 2022.
Credit: Source link
Comments are closed.