Microsoft Research Introduces ‘Tutel’: A High-Performance MoE Library To Facilitate The Development Of Large-Scale DNN (Deep Neural Network) Models

‘The Mixture of Experts (MoE) architecture is a deep learning model architecture in which the computational cost is proportional to the number of parameters, allowing for simpler scaling’. MoE is currently the only approach that has been demonstrated to scale deep learning models to trillions of parameters, paving the way for models capable of learning even more information and powering computer vision, speech recognition, natural language processing, and machine translation systems, among other applications that can help people and organizations in new ways.
Tutel is a high-performance MoE library developed by Microsoft researchers to aid in the development of large-scale DNN (Deep Neural Network) models; Tutel is highly optimized for the new Azure NDm A100 v4 series, and Tutel’s diverse and flexible MoE algorithmic support allows developers across AI domains to execute MoE more easily and efficiently. Tutel achieves an 8.49x speedup on an NDm A100 v4 node with 8 GPUs and a 2.75x speedup on 64 NDm A100 v4 nodes with 512 A100 GPUs compared to state-of-the-art MoE implementations like Meta’s Facebook AI Research Sequence-to-Sequence Toolkit (fairseq) in PyTorch for a single MoE layer.
Tutel delivers a more than 40% speedup for Meta’s 1.1 trillion–parameter MoE language model with 64 NDm A100 v4 nodes for end-to-end performance, thanks to optimization for all-to-all communication. When working on the Azure NDm A100 v4 cluster, Tutel delivers exceptional compatibility and comprehensive capabilities to assure outstanding performance. Tutel is free and open-source software that has been integrated into fairseq.
Tutel is a high-level MoE solution that complements existing high-level MoE solutions like fairseq and FastMoE by focusing on the optimizations of MoE-specific computation and all-to-all communication and other diverse and flexible algorithmic MoE supports. Tutel features a straightforward user interface that makes it simple to combine with other MoE systems. Developers can also use the Tutel interface to include independent MoE layers into their own DNN models from the ground up, taking advantage of the highly optimized state-of-the-art MoE features right away.
MoE-based DNN models rely on a naive combination of numerous off-the-shelf DNN operators supplied by deep learning frameworks such as PyTorch and TensorFlow to assemble the MoE computation due to a lack of efficient implementations. Due to redundant computing, such a method results in considerable performance overheads. Tutel creates and implements many GPU kernels that provide operators for MoE-specific calculations. Tutel, for example, reduces the temporal complexity of dispatching “gating output” from O(N3) to O(N2), improving data dispatching efficiency dramatically. Tutel additionally uses a fast cumsum-minus-one operator, which speeds up the process by 24 times over fairseq.
Tutel optimizes all-to-all collective communication on Azure NDm A100 v4 clusters for large-scale MoE training, including CPU-GPU binding and adaptive routing (AR) adjustment. On a multi-non-uniform memory access (NUMA) system, effective CPU-GPU binding is crucial for all-to-all performance, notably on the NDm A100 v4 nodes. Unfortunately, present machine learning frameworks lack an efficient all-to-all communication library, resulting in large-scale distributed training performance regression. Tutel automatically optimizes the binding and provides an intuitive interface for fine-tuning by the user. Tutel also uses multipath technology, specifically AR, on NDm A100 v4 clusters. The total data traffic size of the communication for each GPU does not change in MoE’s all-to-all communication, but the data size between each GPU pair shrinks as the number of GPUs grows.
On Azure NDm A100 v4, Meta has been using Tutel to train its large language model, which uses an attention-based neural architecture akin to GPT-3. The model consists of 32 layers of attention, each with 32 x 128-dimension heads. One MoE layer is present in every two layers, and each GPU has one expert. SInce all-to-all communication becomes the bottleneck as the number of GPUs increases, Tutel gains up to 131 percent with 8 A100 GPUs to 40 percent with 512 A100 GPUs. In the next version, more optimizations are expected.
Conclusion
MoE is a technology that has a lot of potentials. It allows for holistic training using approaches from a variety of fields, such as systematic routing and network balancing with large nodes, and it can even take advantage of GPU-based acceleration. Tutel outperformed the fairseq framework and has since been incorporated into the DeepSpeed framework. Tutel and related connections will help Azure services, particularly for companies looking to scale huge models easily. Tutel will continue to evolve and offer more exciting outcomes, as the MoE is still in its early phases and more efforts are required to realize its full potential.
Github: https://github.com/microsoft/tutel
Reference: https://www.microsoft.com/en-us/research/blog/tutel-an-efficient-mixture-of-experts-implementation-for-large-dnn-model-training/
Suggested
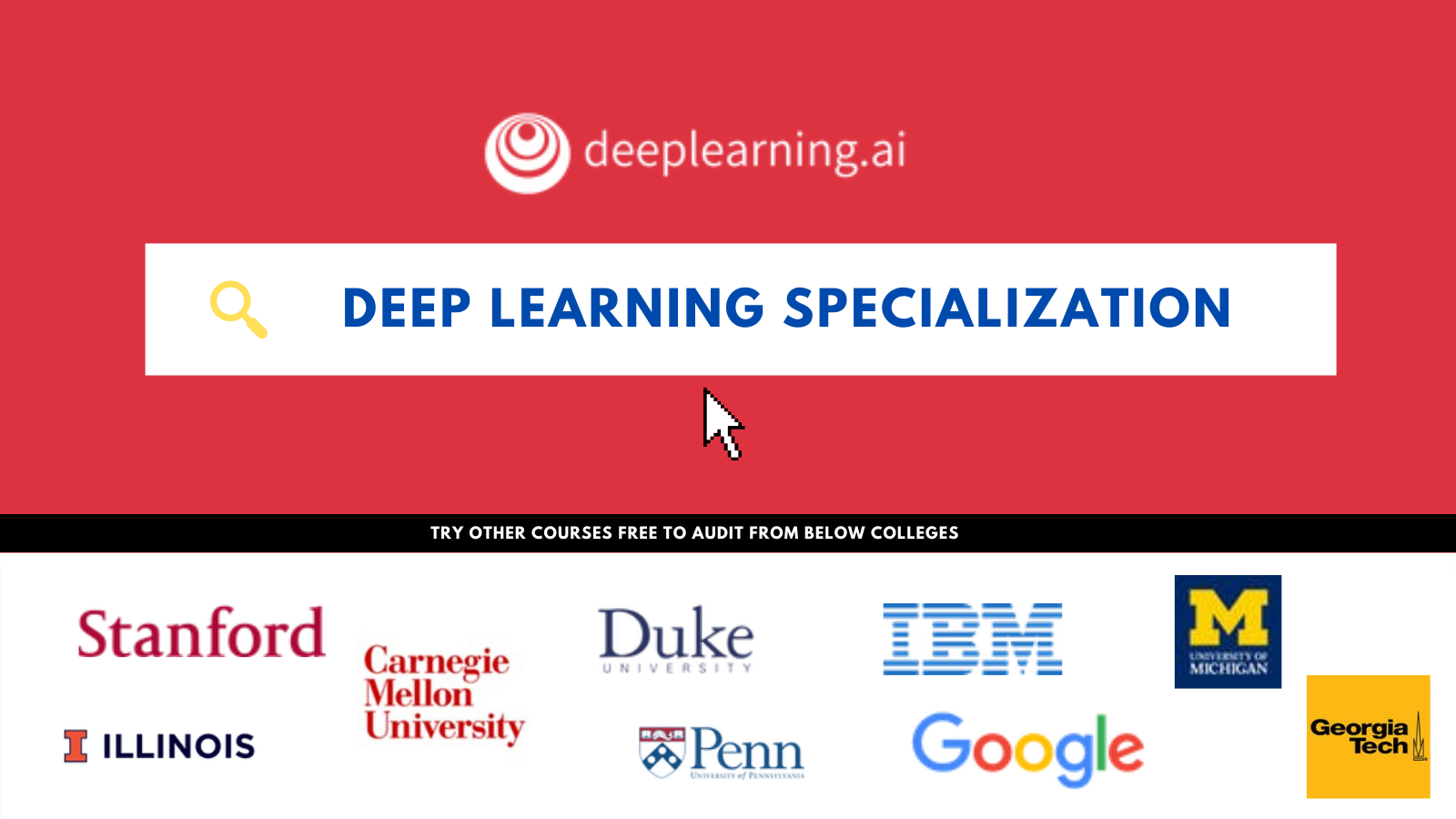
Credit: Source link
Comments are closed.