A Team of AI Researchers Propose ‘GLIPv2’: a Unified Framework for (VL) Vision-Language Representation Learning that Serves Both Localization Tasks and VL Understanding Tasks
This Article is written as a summary by Marktechpost Staff based on the article 'TaiChi: Open Source Library for Few-Shot NLP'. All Credit For This Research Goes To Researchers on This Project. Checkout the paper and github Please Don't Forget To Join Our ML Subreddit
With breakthroughs in object identification and recognition, understanding the context of items in a picture has become increasingly crucial. For example, an umbrella and a human are recognized in an image, and it is helpful to know if the person is carrying the umbrella. Finding solutions to these problems has heightened public interest in developing general-purpose vision systems.
General-purpose vision systems, also known as vision foundation models, tackle several vision tasks simultaneously, such as picture categorization, object identification, and Visual-Language (VL) comprehension. The integration of localization tasks like object identification, segmentation, and VL comprehension is of particular relevance (e.g., VQA and image captioning). A long-standing difficulty is the integration of localization and comprehension, which strives for mutual benefit, a streamlined pre-training method, and lower pre-training costs. However, these two types of tasks appear to be very different: localization tasks are vision-only and need fine-grained output (e.g., bounding boxes or pixel masks), whereas VL understanding tasks stress fusion of two modalities and require high-level semantic outputs (e.g., answers or captions).
Researchers before this study attempted to integrate these tasks in a basic multi-task approach, where a low-level visual encoder is shared across tasks, and two different high-level branches are created for localization and VL comprehension, respectively. Localization tasks are still vision-only and do not take advantage of the rich semantics in vision-language data.
In this study, “VL grounding” is identified as a skill for localization and comprehension. VL grounding entails comprehending an input language and locating the items referenced in the picture (check Figure 1). As a unified model for localization and VL understanding tasks, a grounded VL understanding model (GLIPv2) is constructed.
Localization + VL comprehension = grounded VL comprehension. Localization challenges entail localization and semantic classification, where classification may be framed as a VL understanding issue using the classification-to-matching method. Localization data are converted into VL grounding data as needed. The vast VL understanding data (image-text pairings) may be simply self-trained into VL grounding data. As a result, GLIPv2 includes a unified pre-training procedure in which all task data are converted to grounding data, and GLIPv2 is pre-trained to perform grounded VL comprehension.
Inter-image region-word contrastive learning is a more powerful VL grounding challenge. As its pre-training assignment, GLIP recommends the phrase grounding task, which we contend is a simple challenge that does not correctly use data information.
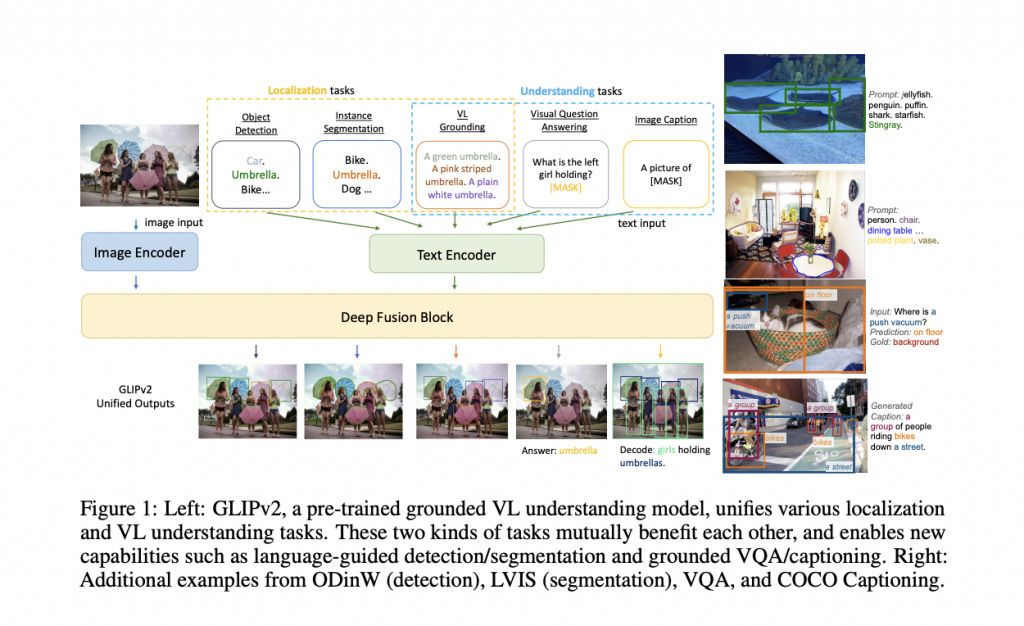
In Figure 1, for example, the phrase grounding challenge merely needs the model to match a given picture region to one of three phrases in the text input, i.e., “green, pink striped, or plain white umbrella?” This 1-in-3 option is relatively simple, requiring only color comprehension, but it loses much information in the grounding data: the umbrellas are not other colors, such as black, yellow, and so on; the objects in those regions are umbrellas but not other categories, such as automobile, bike.
GLIPv2 provides reciprocal gain from localization and VL comprehension.
1) Experimental findings reveal that a single GLIPv2 model produces near SoTA performance on various localization and comprehension tasks.
2) GLIPv2 demonstrates improved zero-shot and few-shot transfer learning capacity to open-world object recognition and instance segmentation tasks on the LVIS dataset and the “Object Detection in the Wild (ODinW)” benchmark, thanks to semantic-rich annotations from image-text data.
3) GLIPv2 supports language-guided identification and segmentation, with new SoTA performance on the Flick30K-entities phrase two grounding and PhraseCut referencing image segmentation tasks.
4) Because GLIPv2 is inherently a grounding model, it produces VL understanding models with excellent grounding capabilities that are self-explainable and easy to debug. For example, GLIPv2 may answer questions while localizing stated things when finetuned on VQA
The code is accessible on GitHub, and the demo is available on a Colab notebook.
Promote Your Brand 🚀 Marktechpost – An Untapped Resource for Your AI/ML Coverage Needs
Get high-quality leads from a niche tech audience. Benefit from our 1 million+ views and impressions each month. Tap into our audience of data scientists, machine learning researchers, and more.
Credit: Source link
Comments are closed.