A research collaboration, including contributors from NVIDIA and MIT, has developed a machine learning method that can identify hidden people simply by observing indirect illumination on a nearby wall, even when the people are nowhere near the illuminating light sources. The method has an accuracy near to 94% when attempting to identify the number of hidden people, and can also identify the specific activity of a hidden person by massively amplifying light bounces that are invisible to human eyes and to standard methods of image amplification.
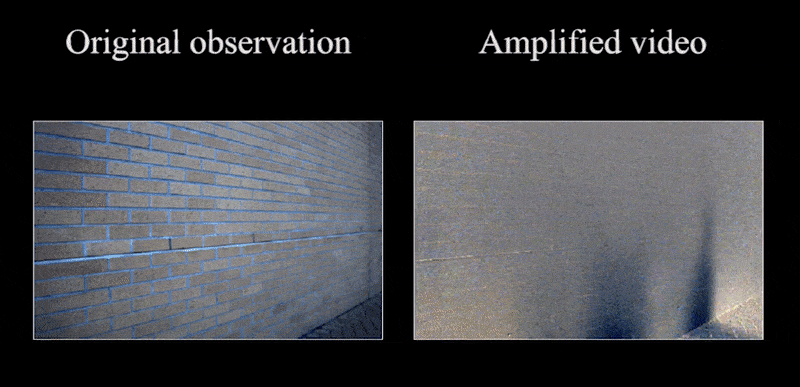
Imperceptible perturbations of light, amplified by the new method, which uses convolutional neural networks to identify areas of change. Source: https://www.youtube.com/watch?v=K4PapXyX-bI
The new paper is titled What You Can Learn by Staring at a Blank Wall, with contributions from NVIDIA and MIT, as well as the Israel Institute of Technology.
Prior approaches to ‘seeing around walls’ have relied on controllable light sources, or prior knowledge of known sources of occlusion, whereas the new technique can generalize to any new room, with no requirement for recalibration. The two convolutional neural networks that individuate hidden people used data obtained from only 20 scenes.
The project is aimed at high-risk, security-critical situations, for search and rescue operations, general law enforcement surveillance tasks, emergency response scenarios, for fall detection among elderly people, and as a means to detect hidden pedestrians for autonomous vehicles.
Passive Evaluation
As is frequently the case with computer vision projects, the central task was to identify, classify and operationalize perceived state changes in an image stream. Concatenating the changes leads to signature patterns that can be used either to identify a number of individuals or to detect the activity of one or more individuals.
The work opens up the possibility of completely passive scene evaluation, without the need to utilize reflective surfaces, Wi-Fi signals, radar, sound or any other ‘special circumstances’ required in other research efforts of recent years that have sought to to establish hidden human presence in a hazardous or critical environment.

A sample data-gathering scenario of the type used for the new research. The subjects are carefully positioned not to cast shadows or to directly occlude any lights, and no reflective surfaces or other ‘cheat’ vectors are permitted. Source: https://arxiv.org/pdf/2108.13027.pdf
Effectively, the ambient light for the typical scenario envisaged for the application would overwhelm any minor perturbations caused by reflected light from people hidden elsewhere in the scene. The researchers calculate that the light-disturbance contribution of the individuals would typically be less than 1% of the total visible light.
Removing Static Lighting
In order to extract motion from the apparently static wall image, it’s necessary to calculate the temporal average of the video and remove it from each frame. The resulting patterns of movement are usually below the noise threshold of even good-quality video equipment, and in effect much of the movement occurs within a negative pixel space.
To remedy this, the researchers downsample the video by a factor of 16 and upscale the resulting footage by a factor of 50, whilst adding a middle-gray base level to discern the presence of negative pixels (which could not be accounted for by baseline video sensor noise).
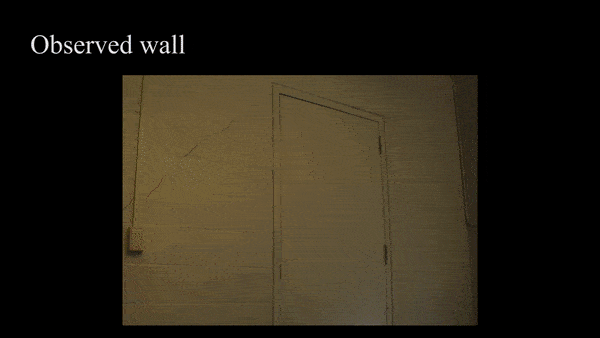
The difference between the human-perceived wall, and the extracted perturbation of hidden individuals. Since image quality is a central issue in this research, please refer to the official video at the end of the article for a higher-quality image.
The window of opportunity to perceive movement is very fragile, and can be affected even by the flickering of lights at a 60 Hz AC frequency. Therefore this natural perturbation also has to be evaluated and removed from the footage before person-induced movement will emerge.
Finally, the system produces space-time plots that signal a specific number of hidden room inhabitants – discrete visual signatures:

Signature space-time plots representing different numbers of hidden people in a room.
Different human activities will also result in signature perturbations which can be classified and later recognized:

The space-time plot signatures for inactivity, walking, crouching, waving hands, and jumping.
In order to produce an automated machine learning-based workflow for hidden person recognition, varied footage from 20 apposite scenarios was used to train two neural networks operating on broadly similar configurations – one to count the number of people in a scene, and the other to identify any movement occurring.
Testing
The researchers tested the trained system in ten unseen real-world environments designed to recreate the limitations anticipated for ultimate deployment. The system was able to achieve up to 94.4% accuracy (over 256 frames – typically just over 8 seconds of video) in classifying the number of hidden people, and up to 93.7% accuracy (under the same conditions) in classifying activities. Though accuracy drops with fewer source frames, it’s not a linear drop, and even 64 frames will achieve a 79.4% accuracy rate for ‘number-of-people’ evaluation (against nearly 95% for four times the number of frames).
Though the method is robust to weather-based changes in lighting, it struggles in a scene illuminated by a television, or in circumstances where the people are wearing monotone clothing the same color as the reflecting wall.
More details of the research, including higher-quality footage of the extractions, can be seen in the official video below.
Credit: Source link
Comments are closed.