The role of diversity has been a subject of discussion in various fields, from biology to sociology. However, a recent study from North Carolina State University’s Nonlinear Artificial Intelligence Laboratory (NAIL) opens an intriguing dimension to this discourse: diversity within artificial intelligence (AI) neural networks.
The Power of Self-Reflection: Tuning Neural Networks Internally
William Ditto, professor of physics at NC State and director of NAIL, and his team built an AI system that can “look inward” and adjust its neural network. The process allows the AI to determine the number, shape, and connection strength between its neurons, offering the potential for sub-networks with different neuronal types and strengths.
“We created a test system with a non-human intelligence, an artificial intelligence, to see if the AI would choose diversity over the lack of diversity and if its choice would improve the performance of the AI,” says Ditto. “The key was giving the AI the ability to look inward and learn how it learns.”
Unlike conventional AI that uses static, identical neurons, Ditto’s AI has the “control knob for its own brain,” enabling it to engage in meta-learning, a process that boosts its learning capacity and problem-solving skills. “Our AI could also decide between diverse or homogenous neurons,” Ditto states, “And we found that in every instance the AI chose diversity as a way to strengthen its performance.”
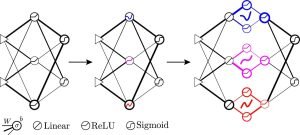
Progression from conventional artificial neural network to diverse neural network to learned diverse neural network. Line thicknesses represent weights
Performance Metrics: Diversity Trumps Uniformity
The research team measured the AI’s performance with a standard numerical classifying exercise and found remarkable results. Conventional AIs, with their static and homogenous neural networks, managed a 57% accuracy rate. In contrast, the meta-learning, diverse AI reached a staggering 70% accuracy.
According to Ditto, the diversity-based AI shows up to 10 times more accuracy in solving more complex tasks, such as predicting a pendulum’s swing or the motion of galaxies. “Indeed, we also observed that as the problems become more complex and chaotic, the performance improves even more dramatically over an AI that does not embrace diversity,” he elaborates.
The Implications: A Paradigm Shift in AI Development
The findings of this study have far-reaching implications for the development of AI technologies. They suggest a paradigm shift from the currently prevalent ‘one-size-fits-all’ neural network models to dynamic, self-adjusting ones.
“We have shown that if you give an AI the ability to look inward and learn how it learns it will change its internal structure — the structure of its artificial neurons — to embrace diversity and improve its ability to learn and solve problems efficiently and more accurately,” Ditto concludes. This could be especially pertinent in applications that require high levels of adaptability and learning, from autonomous vehicles to medical diagnostics.
This research not only shines a spotlight on the intrinsic value of diversity but also opens up new avenues for AI research and development, underlining the need for dynamic and adaptable neural architectures. With ongoing support from the Office of Naval Research and other collaborators, the next phase of research is eagerly awaited.
By embracing the principles of diversity internally, AI systems stand to gain significantly in terms of performance and problem-solving abilities, potentially revolutionizing our approach to machine learning and AI development.
Credit: Source link
Comments are closed.