Kimberly Powell is vice president of healthcare at NVIDIA. She is responsible for the company’s worldwide healthcare business, including hardware and software platforms for accelerated computing, AI, and visualization that power the ecosystem of medical imaging, life sciences, drug discovery, and healthcare analytics. Previously, Powell led the company’s higher education and research business, along with strategic evangelism programs, NVIDIA AI Labs, and the NVIDIA Inception program with over 8,500 AI startup members. Powell joined NVIDIA in 2008 with the responsibility for establishing NVIDIA GPUs as the accelerator platform for medical imaging instruments.
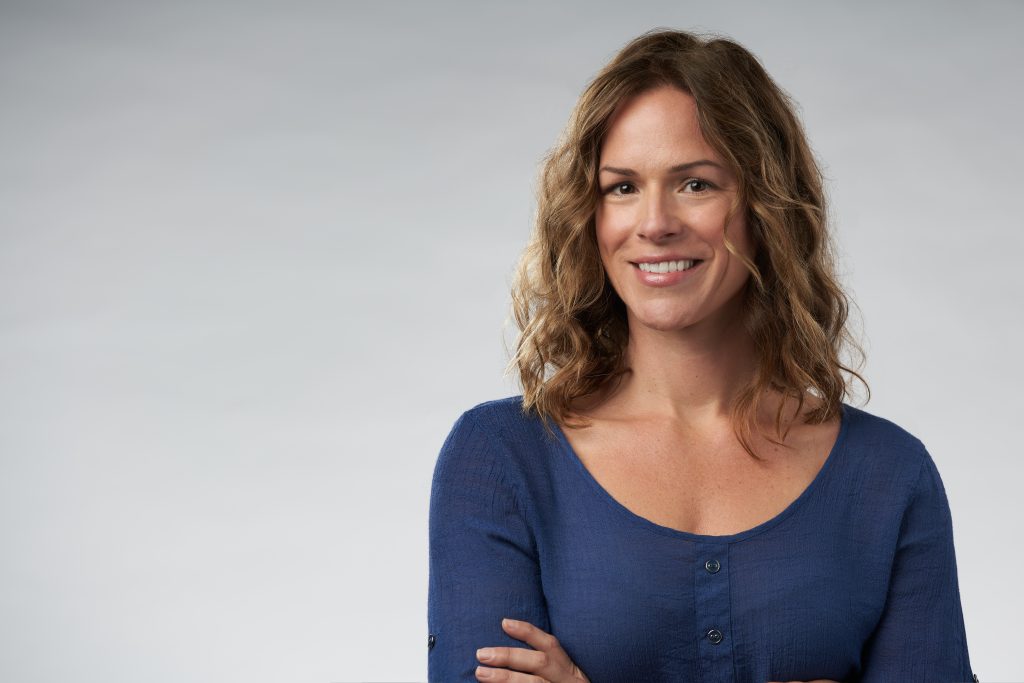
Q1. Tell us about your journey at NVIDIA. How has the advent of the NVIDIA GPU transformed the application of AI in healthcare?
Kimberly: My journey at NVIDIA started 14 years ago in the medical devices sector. When I started, NVIDIA was primarily known for computer graphics, and over time, NVIDIA has expanded into other areas, including supercomputing and artificial intelligence.
GPU & Computer Graphics
NVIDIA’s foundational invention was the graphics processing unit (GPU). The GPU’s purpose is a very high-level, parallel processing unit to run certain applications at orders of magnitude faster than CPUs or other architectures. The GPU is what really got me excited about joining the company when I did about 14 years ago. This type of invention creates paradigm shifts in industries.

The first killer application of GPUs was computer graphics. In fact, our first application in healthcare was for computer graphics and radiology. Radiology is a field where we use devices to see inside the human body. We wanted to be able to see things in more and more detail, with advanced imaging like in 3D MRI.
Accelerated Computing & Supercomputers
About fifteen years ago, NVIDIA expanded beyond computer graphics into an accelerated computing company. GPU acceleration was paramount for the world’s supercomputers. Supercomputing is an area that we are still heavily involved in today. NVIDIA is powering over 70% of supercomputers, which is pretty incredible.
One of the most important application areas of supercomputing centers globally is Life Sciences. One of the greatest challenges of humanity is to understand disease. At NVIDIA, we do that through very large-scale bioinformatics, molecular modeling, and simulation. This is the tip of the spear of what you could imagine industries like the pharmaceutical industry taking on. We always engage at that whole ecosystem level, starting at research, so that we can be at the bleeding edge of what our industry is going to look like in 5 to 10 years.
Domain-Specific Artificial Intelligence
Now, in 2022, the biggest and fastest-growing application area is artificial intelligence. AI is going beyond graphics in terms of what it’s doing for our company. It is the biggest technology force of the current time. We have always firmly believed that. Now the mission statement of NVIDIA Healthcare is to bring that capability of artificial intelligence to the healthcare industry.
If you think about AI and the notion of intelligence, it means that it’s domain-specific. There is a reason why doctors go to school and practice for decades before they are considered a specialist; because it’s very domain-specific. That is what we are doing in the healthcare industry. We are taking these computational approaches that NVIDIA has pioneered from computer graphics to accelerated computing and artificial intelligence and putting them in the hands of the healthcare industry.
Back 14 years ago, when I started the healthcare practice for NVIDIA, we were getting all these early indicators saying that this sensor technology that was being invented needed a step function in terms of its computing power. All these improvements in the sensor technology put this huge strain on the downstream processing and the human interpretation of all of that data. Today, you will see NVIDIA inside of all of your modern medical devices, including CT, MRI, ultrasound, genomic sequencers, and microscopes.
Artificial intelligence is becoming the computational workhorse for medical device innovation. It is an area that NVIDIA is really, really focused on and we have built computing platforms to support this.
Q2. How would you describe NVIDIA’s role in contributing to the ecosystem of medical imaging, life sciences, drug discovery, and healthcare analytics?
Kimberly: Healthcare is a major industry. Those are four segments of the healthcare ecosystem that are also giant in nature. NVIDIA started in medical devices and it is still one of our core areas of contribution. The nature of medical device innovation has all of this high throughput data, and this is what is triggering the digital biology revolution. Genomics is one of the most intense data science areas ever because of these 3 billion letters that make up the story of each individual human.
NVIDIA has this unique view of being a computing platform company. We think about medical devices and allowing them to become more sophisticated in what they can sense and what they can build into their sensors. We want to help the medical device sector innovate. By helping the healthcare industry in creating all of this downstream data to really understand human disease, and then applying it to the challenges in each one of these industries.
Drug Discovery & Genomics
In drug discovery, you think about what steps need to be taken. We first have to identify the target, then identify what molecules might affect the behavior of that target protein, then tie that all together in ways that are completely in silico (completely done in the computer). We use artificial intelligence, modeling, and simulation so we can reduce the amount of expensive time-consuming, error-prone experimentation that has been been used previously in early-stage drug development. You look at genomics being at the front of that pipeline, to really teach us about and help identify the genes that code for the proteins that cause our body to do certain things – good and bad. And then all the way through to these very, very large simulation problems. If you look at the drug discovery process that encapsulates it all: We go through genomics and proteins and molecular simulations, all the way through to clinical trials. NVIDIA is instrumental throughout the process- from identifying the genes and variants, all the way to early-stage in silico drug development, finishing with clinical trials.
Doing Things In Silico
How is this manifesting inside of an actual human, studying that and bringing it all back again and creating this sort of loop? At NVIDIA we are trying to, as much as we can, put the ecosystem and processes in silico. Computer science approaches – whether it be scientific computing, artificial intelligence, or advanced visualization technique – can be applied to this data in new and sophisticated ways. This data processing is well beyond what a human, any one human, could really endure, take on, and make decisions about. So doing things in silico is really how we think about it.
Q3. Can you tell us a little about NVIDIA Clara and other healthcare tools that NVIDIA is working on? How are these tools impacting elements of the healthcare industry, such as radiology, medical imaging, and genomics?
Kimberly: NVIDIA is a whole stack computing company. This has really helped the pharmaceutical industry understand us a bit more. Some people just call us a chip company, and we obviously find that really flawed with all the work that we are doing now.
Layer 1: GPU, chips, systems, data centers
The first layer of the stack is really around the GPU. However, we have also moved well into full-on systems. That first layer is about chips, systems, and whole data centers as our product.
Layer 2: Acceleration Layer
The second layer is the acceleration layer and is where the acceleration comes in. How do you take advantage of that architecture at more than a chip-level? At multiple chip levels? At multiple node levels? That acceleration layer is what really put NVIDIA on the map in the area of artificial intelligence, being able to do this deep learning at very, very large scale. A lot of the things we build at this second layer can be used in financial services, in autonomous vehicles, in the omniverse.
Layer 3: Industry Application Framework Layer
In these last 5 to 10 years we are developing the third layer of our company, and we call that the industry application framework layer. The goal of the third layer is to take that acceleration and system layer, and make it more domain-specific.
For the healthcare domain, we call that industry application framework NVIDIA Clara. This framework was named after Clara Barton, the inventor of the American Red Cross, and we think of Clara as a platform to help people. Our Clara platform builds upon those first two layers below it – the GPU system layer and data center layer – and leverages everything we do, as a 20,000 plus company. In this third layer of Clara, we focus on a few very specific areas.
Clara Holoscan:
Medical devices are our core. Over the last several years, we have developed our Software-Defined computational platform for medical devices called Clara Holoscan. This is where we are building actual specific systems so medical devices can do the end-to-end workloads that they need: everything from very high throughput sensor processing, to all of the AI processing they want to do in-device, and even doing visualization. Think about an ultrasound machine: You have a sensor in and display out all on the same machine, and all of the AI and image processing that has to happen in between. This is a very typical pipeline for medical devices.
We are building a computational platform so that medical device manufacturers will not have to think about the nuts and bolts in this. It really builds upon NVIDIA’s three core engines that we have now. (1) We invented the GPU. (2) We have been pioneering ARM-based processors. Our ARM CPU architecture is what powers all of our self-driving but now can be used for things like medical devices. (3) The third one we recently added to our family with the Mellanox acquisition is our data processing unit (DPU). You need to get data into the node at very, very high speeds. So we now have this three-engine architecture. And that’s, again, a very unique position of NVIDIA.
We want to make it much easier for the medical device community to take advantage of those three engines, and to really help them accelerate their innovation in that space. So Clara Holoscan is exactly that. The system architecture to the acceleration layer that sits on top of that to the domain-specific applications. If you look at Clara Holoscan, we have reference applications for endoscopy which is what is powering this minimally invasive surgical market. Clara Holoscan is one of the places that we are really, really excited about, and you are going to see a lot of upcoming development in that area.
We want these medical devices to become a self-driving car in a sense. What do I mean by that? We want to move into the software-as-a-service business model. Companies do not want to sell an instrument once and have to maintain it for 10 years. They want to be able to continue to innovate on AI applications and increase value upon the instrument that goes in it. That computational platform we built allows for that software-defined, medical device era to come to this field. Much of what we have learned from self-driving is completely applicable to the medical devices market.
NVIDIA Clara Discovery
Another area that we are greatly focused on is taking all of the work that we do in the supercomputing industry, and everything that we have learned from artificial intelligence, and bringing it into the drug discovery market. In the last 18 months, we announced NVIDIA Clara Discovery, our computational platform for drug discovery. Clara Discovery is all about the bleeding edge of AI and applying it to this very unique data within medical devices and biotech. We are looking at data such as protein sequence data or smile strings that represent a molecule in the bioinformatics space and genomics data.
Transformers & Generative Models
I saw that Marktechpost followed transformers and generative models in 2021 and how applicable they are to these incredibly challenging datasets. Alphafold 2, enabled by transformer AI, allows you to essentially feed whole databases of protein sequence information so you can predict the structure of a protein.
We are pioneering generative models for molecule generation with AstraZeneca using something we call MegaMoIBART. Using transformers in generative models to go beyond the molecular databases that exist, because there are 10^60 potential molecules that we could build. Our databases are still quite small and we want to be able to explore as much as we can. It has a lot of downstream applications in the drug discovery space.
MONAI & Computer Vision
I think most people know NVIDIA in the healthcare space on the imaging side. What we have done over the last four years is build an AI framework for medical AI. We call this framework the Medical Open Network for Artificial Intelligence, or MONAI. MONAI is a PyTorch-based framework for deep learning in healthcare imaging. MONAI is largely targeting a lot of the imaging applications – such as radiology, pathology, or real-time video – used in the surgical space. This week (January 19, 2022), we surpassed 200,000 downloads of this framework. It has all the domain-specific data ingestion, transformation, and model architectures used in this space.
How do you deploy this into a clinical environment so you can validate it? We are working with a huge consortium of contributors with MONAI and building this application framework because we want to make it very, very accessible.
Computer Vision was one of the frontrunners of the application of AI. Computer vision applications in healthcare have tremendous opportunities. Tens of thousands of algorithms will be developed to serve the radiology industry. You can use algorithms everywhere from capturing the right image to de-noising that image to then looking for anatomical structures in that image. Doing all of the things that are repetitive that humans have to do and then presenting that information such that we can help the overstretched radiologists. With MONAI, we are really excited about what’s happening in that space and we continue to put a lot of focus on that.
Data access & federated learning
Secondary to MONAI and just building the applications, we are also addressing one of the main challenges in healthcare around data access. A lot of computer vision and CNN (convolutional neural networks) approaches require a lot of data, a lot of labeled data. MONAI helps with that. Healthcare data changes all the time. We want to enable a world that can better adapt to that. All of a sudden, we are seeing lungs that have COVID pneumonia that we have never seen before. How can we create robust algorithms in real-time for that? We are doing that through a federated learning platform.
NVIDIA FLARE
We recently announced open-source NVIDIA FLARE (Federated Learning Application Runtime Environment), which is our federated learning framework. We worked with a consortium of 20 different hospitals and a model that was developed at Mass General. We delivered that model to 20 different hospitals so they could contribute learnings from their data but not have to contribute any data at all. It created this really amazing multi-role model that predicts the oxygen need of a patient who had an x-ray and had some lab work done. It shows that the future of AI development will be in a federated manner. Federated learning allows you to learn from data that is happening out on the edge but to not have to share that data that’s happening on the edge.
So that in a nutshell is a lot of what Clara is focused on. We also have lots of efforts in NLP but, there is probably more than we can touch on.
Q4. How is NVIDIA planning to use federated learning within its healthcare division?
Kimberly: With open-source NVIDIA FLARE (Federated Learning Application Runtime Environment), we work with a lot of collaborators. At Mass General, they had this really neat model that used two different modality types: (1) electronic health record data and (2) X-ray data. The two different modality types combined to make this prediction. After we did this program, we actually package all of the training tools and the model itself into our NGC, which is essentially our AI software hub. We publish the model so that the world can take and build upon it. It is not an FDA-approved algorithm, but it is meant to be a tool to help the world build upon it: whether they want to learn how to do federated learning, or whether they actually want to take that model and build it into their own application framework to go through the FDA validation process. We see this as absolutely the future of model co-development.
NVIDIA is getting approached by a lot of the industry to do that co-development. This is a very safe way to respect the privacy of data, but move the field forward and develop cutting-edge algorithms that can be heavily used. We are also enabling all of our other industries, whether it be our self-driving car industry, our financial services industry, or our retail industry. All industries have data governance challenges. Data cannot be static. Data isn’t static. If you want AI to be able to deal with non-static data, it has to learn from non-static data. We believe federated learning is absolutely going to be what call AI 2.0. Federated learning will allow us to be able to take advantage of all the data that the world is going to continuously produce in a safe way.
Q5. Tell us about some of NVIDIA’s latest partnerships in healthcare and AI.
Cambridge-1
We have many partnerships going on. One of the ones that we are super excited about is built in the UK, called Cambridge-1. Cambridge-1 is dedicated to large-scale AI research in healthcare and the first NVIDIA built for external access. One of our collaborators, Kings College London, has developed some brain disease algorithms on it and they are actually deploying it into their clinical environment. We are also working with startups like Peptone, and others on Cambridge-1, which is the most powerful AI supercomputer in the UK.
We are also working with AstraZeneca on these transformer-based generative models for molecule generation, which we call MegaMoIBART.
Genomics & Oxford Nanopore Technologies
We are working with the genomics sequencing company, Oxford Nanopore Technologies. Stanford University’s Dr. Euan Ashley, had a dream of being able to more rapidly diagnose critical care patients through the use of genomic sequencing. Oxford Nanopore Technologies and NVIDIA have been working together for many years. By accelerating the whole pipeline – everything from the base calling on the sequencer all the way through to the variant calling that can decide which genetic disease you may be suffering from – we can more effectively intervene with treatment. We were able to take the world record from 14 hours down to seven and a half hours. NVIDIA is working on the accuracy and the speed of genomics with Oxford Nanopore.
Global Cancer Research
Some of the other partnerships from GTC Fall include cancer research. There is amazing work that the global cancer centers are now doing with AI. There are so many unmet needs in diseases and cancer is a big one of them. We are working with MD Anderson, St. Jude, Memorial Sloan Kettering, and German Cancer Research Center DKFZ.
Working with the startup community
In the last two years, some $40 billion in funding have flown into the drug-discovery startup community, and for good reason. The breakthroughs of alpha folds and protein structure prediction, the advancement of genomics, the fact that we can do more with AI and natural language processing. It’s a perfectly ripe time for these new companies to be established. JP Morgan Health just finished up. There’s just partnership upon partnership of large pharma, partnering with these AI platform companies to really look for advances and acceleration.
Q6. What do you foresee as the biggest challenges in 2022 onward for the AI and healthcare domain and where do you see NVIDIA fitting in?
Kimberly: A couple of challenges that come to mind revolve around innovation, complexity, ease of use, accessibility, and the development of specific tools that address some of the challenging data problems in healthcare. Can Clara Holoscan address the innovation problems? Can all of Clara address the full-stack computing that is complex, but make it easy to use? Can we make it more accessible and state-of-the-art? Can we build specific tools and platforms that address some of the challenging data problems in healthcare?
(1) Reducing the complexity for the healthcare industry as computing complexity continues to grow. In the healthcare sector, mastering the industry involves understanding the clinical problems, the workflows of the doctors, and the patients that they are trying to serve. It is very hard to do that extremely well and stay at the bleeding edge of computing approaches. With Clara Holoscan, we are working to take that complexity and make it very easy for healthcare industry professionals to remain focused on the problems. We want to partner with them on making new computing approaches accessible to them so that:
● Their innovation can be accelerated.
● Their go-to-market can be much easier.
● They can stay innovative by moving into the software-defined, software-as-a-service business model that they so desperately need and want.
How do we reduce the complexity for the healthcare industry so that they can bring these innovations to market sooner? There are all of these AI algorithms, but they have not been productized. There are several reasons for that. We are going to make sure that a computing platform isn’t the reason. You can build this application through our ubiquitous platform. You can deploy it in an instrument. You can deploy it in a data center of the hospital. You can deploy it on any cloud. NVIDIA technology is homogeneous, and you can deploy it where your business model cares to have it.
(2) Being able to stay state of the art and making it easy to do that. NVIDIA wants to make AI accessible for research and discovery. Over in Germany, we are partnered with their cancer center DKFZ so that we can give their clinician / data scientists all the tools to ask as many questions as they wish and build all the AI application models using state-of-the-art approaches.
With MONAI, we are helping doctors use AI to label images to really cut down their time of being the expert, by labeling highly required data. Our computing platforms enable that.
(3) Data accessibility problem
Third is the data accessibility problem. There are several ways you can skin that.
● Federated learning is absolutely one way we can do it. Federated learning is going to be a framework that is going to connect living breathing data to the evolution of models going forward. In the future, federated learning will enable us to develop robust models without sharing data.
● The other is state-of-the-art approaches. What’s so novel about these transformers is you do not do it in a semi-supervised way (you do not have to have labeled data). For healthcare, that is huge because we will never have enough labeled data. We did have some breakthrough research ourselves at NVIDIA. You can use transformers for natural language processing, you can use transformers for pre-construction prediction. We want to use state-of-the-art approaches that help us overcome some of the data challenges.
This interview was originally published in our AI in Healthcare Magazine (March 2022)
Credit: Source link
Comments are closed.