Not all deepfake practitioners share the same objective: the impetus of the image synthesis research sector – backed by influential proponents such as Adobe, NVIDIA and Facebook – is to advance the state of the art so that machine learning techniques can eventually recreate or synthesize human activity at high resolution and under the most challenging conditions (fidelity).
By contrast, the objective of those who wish to use deepfake technologies to spread disinformation is to create plausible simulations of real people by many other methods than the mere veracity of deepfaked faces. In this scenario, adjunct factors such as context and plausibility are almost equal to a video’s potential to simulate faces (realism).
This ‘sleight-of-hand’ approach extends to the degradation of final image quality of a deepfake video, so that the entire video (and not just the deceptive portion represented by a deepfaked face) has a cohesive ‘look’ that’s accurate to the expected quality for the medium.
‘Cohesive’ doesn’t have to mean ‘good’ – it’s enough that the quality is consistent across the original and the inserted, adulterated content, and adheres to expectations. In terms of VOIP streaming output on platforms such as Skype and Zoom, the bar can be remarkably low, with stuttering, jerky video, and a whole range of potential compression artifacts, as well as ‘smoothing’ algorithms designed to reduce their effects – which in themselves constitute an additional range of ‘inauthentic’ effects that we have accepted as corollaries to the constraints and eccentricities of live streaming.
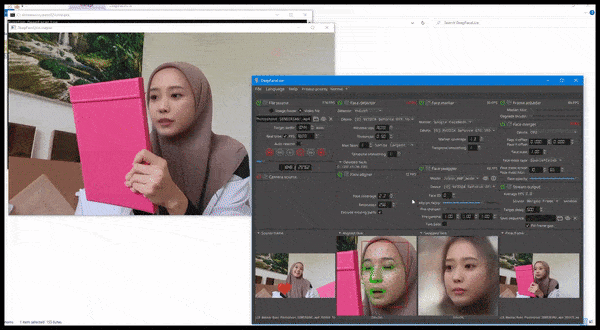
DeepFaceLive in action: this streaming version of premier deepfake software DeepFaceLab can provide contextual realism by presenting fakes in the context of limited video quality, complete with playback issues and other recurrent connection artifacts. Source: https://www.youtube.com/watch?v=IL517EgYH8U
Built-In Degradation
Indeed, the two most popular deepfake packages (both derived from the controversial 2017 source code) contain components intended to integrate the deepfaked face into the context of ‘historical’ or lower-quality video by degrading the generated face. In DeepFaceLab, the bicubic_degrade_power parameter accomplishes this, and in FaceSwap, the ‘grain’ setting in the Ffmpeg configuration likewise helps integration of the false face.

The ‘grain’ setting in FaceSwap aids authentic integration into non-HQ video content, and legacy content that may feature film grain effects that are relatively rare these days.
Often, instead of a complete and integrated deepfake video, deepfakers will output an isolated series of PNG files with alpha channels, each image showing only the synthetic face output, so that the image stream can be converted into video in platforms with more sophisticated ‘degrading’ effects capabilities, such as Adobe After Effects, before the fake and real elements are joined together for the final video.
Besides these intentional degradations, the content of deepfake work is frequently recompressed, either algorithmically (where social media platforms seek to save bandwidth by producing lighter versions of users’ uploads) in platforms such as YouTube and Facebook, or by reprocessing of the original work into animated GIFs, detail sections, or other diversely motivated workflows that treat the original release as a starting point, and subsequently introduce additional compression.
Realistic Deepfake Detection Contexts
With this in mind, a new paper from Switzerland has proposed a revamping of the methodology behind deepfake detection approaches, by teaching detection systems to learn the characteristics of deepfake content when it is presented in deliberately degraded contexts.

Stochastic data augmentation applied to one of the datasets used in the new paper, featuring Gaussian noise, gamma correction, and Gaussian blur, as well as artifacts from JPEG compression. Source: https://arxiv.org/pdf/2203.11807.pdf
In the new paper, the researchers argue that vanguard deepfake detection packages are relying on unrealistic benchmark conditions for the context of the metrics that they apply, and that ‘degraded’ deepfake output can fall below the minimum quality threshold for detection, even though their realistically ‘grungy’ content is likely to deceive viewers due to a correct attention to context.
The researchers have instituted a novel ‘real world’ data degradation process that succeeds in improving the generalizability of leading deepfake detectors, with only marginal loss of accuracy on the original detection rates obtained by ‘clean’ data. They also offer a new assessment framework that can evaluate the robustness of deepfake detectors in real world conditions, supported by extensive ablation studies.
The paper is titled A New Approach to Improve Learning-based Deepfake Detection in Realistic Conditions, and comes from researchers at the Multimedia Signal Processing Group (MMSPG) and the Ecole Polytechnique Federale de Lausanne (EPFL), both based in Lausanne.
Useful Confusion
Prior efforts to incorporate degraded output into deepfake detection approaches include the Mixup neural network, a 2018 offering from MIT and FAIR, and AugMix, a 2020 collaboration between DeepMind and Google, both data augmentation methods that attempt to ‘muddy’ the training material in a way that’s inclined to help generalization.
The researchers of the new work also note prior studies that applied Gaussian noise and compression artifacts to training data in order to establish the boundaries of the relationship between a derived feature and the noise in which it’s embedded.
The new study offers a pipeline that simulates the compromised conditions of both the acquisition process for imaging and the compression and diverse other algorithms that can further degrade image output in the distribution process. By incorporating this real-world workflow into an evaluative framework, it’s possible to produce training data for deepfake detectors that is more resistant to artifacts.

The conceptual logic and workflow for the new approach.
The degradation process was applied to two popular and successful datasets used for deepfake detection: FaceForensics++ and Celeb-DFv2. Additionally, leading deepfake detector frameworks Capsule-Forensics and XceptionNet were trained on the adulterated versions of the two datasets.
The detectors were trained with the Adam optimizer for 25 and 10 epochs respectively. For the dataset transformation, 100 frames were randomly sampled from each training video, with 32 frames extracted for testing, prior to the addition of degrading processes.
The distortions considered for the workflow were noise, where zero-mean Gaussian noise was applied at six varying levels; resizing, to simulate the reduced resolution of typical outdoor footage, which can typically affect detectors; compression, where varied JPEG compression levels were applied across the data; smoothing, where three typical smoothing filters used in ‘denoising’ are evaluated for the framework; enhancement, where contrast and brightness were adjusted; and combinations, where any mix of three of the aforementioned methods were simultaneously applied to a single image.
Testing and Results
In testing the data, the researchers adopted three metrics: Accuracy (ACC); Area Under Receiver Operating Characteristic Curve (AUC); and F1-score.
The researchers tested the standard-trained versions of the two deepfake detectors against the adulterated data, and found them lacking:
‘In general, most of realistic distortions and processing are exceedingly harmful to normally trained learning-based deepfake detectors. For instance, Capsule-Forensics method shows very high AUC scores on both uncompressed FFpp and Celeb-DFv2 test set after training on respective datasets, but then suffers from drastic performance drop on modified data from our assessment framework. Similar trends have been observed with the XceptionNet detector.’
By contrast, the performance of the two detectors was notably improved by being trained on the transformed data, with each detector now more capable of detecting unseen deceptive media.
‘The data augmentation scheme significantly improves the robustness of the two detectors and meanwhile they still maintain high performance on original unaltered data.’

Performance comparisons between the raw and augmented datasets used across the two deepfake detectors evaluated in the study.
The paper concludes:
‘Current detection methods are designed to achieve as high performance as possible on specific benchmarks. This often results in sacrificing generalization ability to more realistic scenarios. In this paper, a carefully conceived data augmentation scheme based on natural image degradation process is proposed.
‘Extensive experiments show that the simple but effective technique significantly improves the model robustness against various realistic distortions and processing operations in typical imaging workflows.’
First published 29th March 2022.
Credit: Source link
Comments are closed.