A good police officer works under the premise that to catch a criminal you need to think like a criminal and predict their next move.
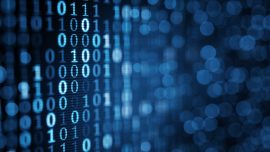
Anomaly detection can model transaction patterns so criminal behaviour stands out
While analysing past behaviour might be successful in catching certain serial offenders, criminals that want to launder money anticipate this way of working and typically will not stick to a pattern.
Still, anti-money laundering (AML) and fraud systems are designed to act like our aforementioned officer. They are set to follow rules based on yesterday’s criminal behaviour while criminals are already making their next move, avoiding patterns and aiming for confusion, dragging risk and compliance professionals into an endless cat and mouse game.
We are seeing a level of sophistication where money trails are obscured by regulatory arbitrage, shell companies and thousands of money mules. All of which are virtually impossible to track down and get ahead of.
While detection engines have got more powerful, they’re still only as good as the models running inside. Building, tuning and deploying these models is still a complex and often cumbersome project with long lead times that don’t match the fluid nature of criminal modus operandi.
A painful result is that 95-99% of reported suspicious activity consists of false positives – transactions that ultimately weren’t illicit but merely a customer making a payment that matched the characteristic of the rules set within the bank. Rules that were set on outdated criminal behaviour.
This begs the question: why chase what you don’t know?
Comparatively speaking, financial institutions don’t have a lot of data on criminals. They only make up a few basis points of their data. And these criminals change their behaviour when they encounter controls.
This is a very challenging reality to model within the timeframe required for effective detection. Turn it around and you see that the vast majority of data is on good customers with good behavior, which doesn’t change so much – or at least not as often as criminal behavior.
Therefore, this behaviour can be modelled more accurately. The big benefit of this approach, called anomaly detection, is that once you understand these patterns of good behavior, criminal patterns clearly stand out. Both known and unknown patterns become visible.
Financial institutions are seeing convincing evidence that this approach is much more effective in detecting financial crime in a customer-friendly manner.
For example, often their most profitable customers are the ones with the most exotic types of transaction patterns. They are therefore also much more likely to experience friction when they encounter a control trying to make a transaction. Once you offset that transaction against this customer’s historical behaviour and that of their peers, you’ll likely conclude that the transaction falls within a pattern of what’s normal for that customer. Think about corporates, by nature of their business, having subsidiaries in other countries, or DJs and rock stars defying time zones traveling all over the world.
However, changing the approach to anomaly detection by focusing on legitimate customer behaviour alone isn’t enough. The largely manual data science process to generate detection models needs to be expedited to keep up with regulation, changes in criminal behaviour and the ongoing shift of channels and business. Deploying anomaly detection based on automated machine learning (AutoML) is one strategy that can be used to improve processes here.
A critical barrier to adoption for AI and machine learning is the required explainability for compliance purposes. The business function of risk (and compliance) has relatively few use cases of adopted AI and machine learning. The vast majority of data science projects fail to move from research to the deployment stage.
In the “machine vs human” challenge, the responsible managers, compliance officers and auditors are often unable to validate the structures of these models to a sufficient level of assurance.
Solutions must enable non-data-scientist decision makers to explain the model to auditors and regulators alike. If a highly complex model succeeds in detecting financial crime, but cannot be explained to these stakeholders, it simply won’t reach production. The best solutions will find the optimal combination of performance and explainability.
Moving from manual approaches to model development with a focus on detecting criminal patterns will be quite a cultural transformation. But it might be the key to solving a problem such as global financial crime.
Credit: Source link
Comments are closed.