Researchers in the UK have proposed a roadside system to automate the detection of illegal mobile phone usage among drivers, using classic photo-optical filters and infra-red capture. Depending on the quality of the capture equipment, the system has demonstrated an accuracy rate of up to 95.81% in real world trials.
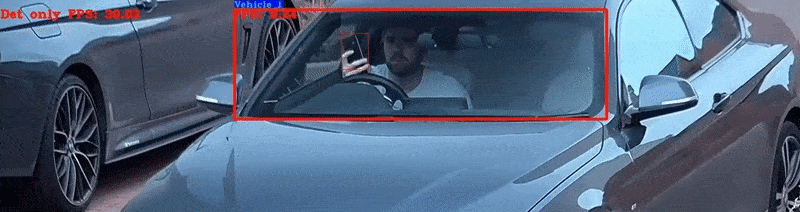
One of the researchers’ models in action. The windshield area is first identified and isolated as a catchment area for an AI-aided search for images of a mobile phone. The system is designed to ignore mounted mobile phones, and seek out devices that are being actively held by the driver. Source: https://www.youtube.com/watch?v=PErIUr3Cxvg
The research is titled Identification of Driver Phone Usage Violations via State-of-the-Art Object Detection with Tracking, and comes from the School of Computing at Newcastle University.
Overcoming Reflectivity of Windshields
Prior approaches to mobile device usage detection among drivers have been hampered by the high reflectivity of windshields during the hours of daylight, exacerbated when reflections from groups of large clouds further obscure the interior of the vehicle. Such cases cannot realistically be addressed with infra-red light sources, since the amount of IR illumination necessary to penetrate natural daylight would be resource-intensive.
Therefore the Newcastle researchers propose the very oldest trick in the book (dating back to 1812) to eliminate reflections from a perceived glass surface – a cheap, physical polarizing filter that could be attached to roadside surveillance cameras, calibrated one time, and thereafter enable a clear look into vehicle interiors.

Above, an unfiltered view of a car windshield. Below, the same view with a physical polarizing filter attached to the camera. Source: https://arxiv.org/pdf/2109.02119.pdf
With the popular move from dedicated cameras to mobile-based sensors, the polarizing filter’s presence in popular culture has largely been reduced to its inclusion in reasonable-quality sunglasses, where the wearer can observe its reflection-killing properties by tilting their viewpoint or changing their viewpoint on the reflective object.
Sunlight is scattered by oxygen and nitrogen molecules, with blue light more extensively scattered than other wavelengths, making blue the native color of a clear sky in daytime. Blue light is polarized, and a linear or circular polarized lens can effectively eliminate this polarized light, removing reflections in the process.
The paper acknowledges that smoked windshields could impede or even foil this method of seeing into the car. However, since this is limited by UK law, with regulations varying by state in the US, the paper does not consider this as a primary obstacle.
YOLO
The system that the paper proposes is intended to be integrated into civic infrastructure, such as government-installed roadside surveillance cameras. Aware of possible obstacles over cost, the researchers tested various object recognition system configurations across a variety of quality-levels of capture equipment, and offer a minimal-cost scenario where cheap polarizing filters could be added to existing cameras, with all other aspects of the system remote.
Four object recognition frameworks were tested: You-Only-Look-Once (YOLO) versions 3 and 4; SSD base network; Faster R-CNN; and CenterNet. In tests, the most accurate results were obtained with YOLO V3, using a two-step workflow which first localizes the windshield area and then seeks a mobile device in that space.
However, the need to run the video through two networks results in a less-than-optimal frame rate of 13.15fps, compared to nearer 30fps on the simpler system. Quality of results depends on the input equipment, and the researchers found that when input was divided between low-end cameras and higher-quality equipment, an accuracy rate of near to 96% was possible on the better kit, and 74.35% on the cheaper cameras.
Limiting Recognized Infractions
In addition to making the system economically viable, the researchers are concerned to develop a fully-automated system with a minimum of necessary human oversight, and the system has been conceived to automatically deliver fines. However, since laws around mobile phone use while driving are becoming more severe around the world, with penalties that may exceed mere fines or license point deductions (i.e. in the UK), it seems likely that casual human verification would remain a factor in deployment of such a system.
In spite of the use of optical flow and other methods to take into account the entirety of video content, object recognition algorithms such as YOLO consider each frame a ‘complete story’, and the next frame a subsequent project. Therefore, a system of this nature must be prevented from issuing (for example) 128 separate fines covering 128 frames of infraction-capturing video.
To avoid this, the system incorporates the object tracking algorithm Deep SORT, which adds a unique ‘incident ID’ to each infraction recognition, and ensures that the ID is not duplicated across frames within a single capture sequence.
Handling Night-Time Surveillance
For night-time conditions, the researchers default to infra-red capture, as used in previous research projects investigating the same challenge. They tested IR wavelengths of 850 and 730 nanometers, and found that the best details were captured with 730nm.
The paper contends that further investigation is necessary to determine to what extent infra-red capture could be used during daytime conditions.
Data
For the more economical single-step version of the system, the researchers used 2,235 license plate images from the Google Open Images Dataset, and 2150 stock and custom-made mobile phone images. Since it was necessary to include images of phones being held by drivers, 1,700 of the phone images were taken specifically for the project.
The two-step system required the annotation of 487 windshields, used to train the first step of the process, in addition to the data used in the one-step process.
Since there was no access to official road surveillance infrastructure, all images were taken by volunteers to approximate similar conditions.
Trade-Offs
The final results offer a range of accuracy standards that would need to be traded off against cost of implementation, with superior capture equipment and processing results offering the greatest accuracy, and arguably ‘acceptable’ accuracy obtainable by inexpensive retro-fitting of existing urban surveillance equipment.

The cheaper, ‘single-step’ pipeline achieves something near to 75% accuracy, with the lowest implementation costs (i.e. the fitting of a cheap polarizing filter), while the more complex two-step system (which isolates the windshield area before seeking a mobile device held by the driver) achieves higher accuracy rates, but may only be suitable for new infrastructure, depending on available budget. In both cases, quality of capture equipment is an additional variable.
As noted above, the researchers’ perception of the viability of the project seems to be informed by the assumption that the system should perform entirely autonomously – a questionable requirement.
Take a look at the project’s official video below for more details on the implementation and approaches used.
Credit: Source link
Comments are closed.