In a Latest Computer Vision Research to Provide Holistic Perception for Autonomous Driving, Researchers Address the Task of LiDAR-based Panoptic Segmentation via Dynamic Shifting Network

One of the most promising uses of computer vision, autonomous driving, has made remarkable development in recent years. One of the most critical modules in autonomous driving, the perception system, has been the subject of much research in the past. To be sure, the traditional tasks of 3D object detection and semantic segmentation have matured to the point where they can support real-world autonomous driving prototypes.
However, there is still a significant gap between current work and the aim of holistic perception, which is critical for complex autonomous driving scenarios. Researchers propose to bridge the gap by investigating the task of LiDAR-based panoptic segmentation, which necessitates full-spectrum point-level predictions, in a recent publication.
In 2D detection, panoptic segmentation has been presented as a new vision task that combines semantic and instance segmentation. Other research expands the challenge to LiDAR point clouds and proposes a LiDAR-based panoptic segmentation task. This challenge requires foreground (things) classes to predict point-level semantic labels, whereas background (stuff) classes (e.g., road, building, and vegetation) require instance segmentation (e.g., car, person, and cyclist).
Despite this, successful panoptic segmentation is challenging due to the complex point distributions of LiDAR data. The majority of existing point cloud instance segmentation approaches were created with dense and uniform indoor point clouds in mind. As a result, the center regression and heuristic clustering algorithms can produce good segmentation results. The center regression, however, fails to give optimum point distributions for clustering due to the non-uniform density of LiDAR point clouds and varied sizes of instances. The regressed centers often create noisy strip distributions with varying densities and widths.
Several heuristic clustering techniques that have been widely employed in past studies are unable to cluster the regression centers of LiDAR point clouds satisfactorily. Researchers suggest the Dynamic Shifting Network (DSNet), which is specifically built for successful panoptic segmentation of LiDAR point clouds, to address the aforementioned technical issues.
To begin, the team selects a strong backbone design and establishes a solid foundation for the new work. In one pass, the cylinder convolution is utilized to extract grid-level features for each LiDAR frame, which are then shared by the semantic and instance branches. Second, the researchers present a new Dynamic Shifting Module that uses complicated distributions formed by the instance branch to cluster on the regressed centers.
The regression centers are shifted to the cluster centers by the suggested dynamic shifting module. The shift targets are calculated adaptively by weighing numerous shift candidates derived using kernel functions. The module’s unique architecture allows the shift operation to dynamically adjust to the density or size of different instances, resulting in better performance on LiDAR point clouds. Further investigation reveals that the dynamic shifting module is stable and unaffected by parameter settings.
Finally, the Consensus-driven Fusion Module is shown, which combines semantic and instance results to produce panoptic segmentation results. The suggested consensus-driven fusion primarily addresses the disagreements produced by the class-agnostic instance segmentation style. Because the fusion module is so efficient, it has very little computational overhead.
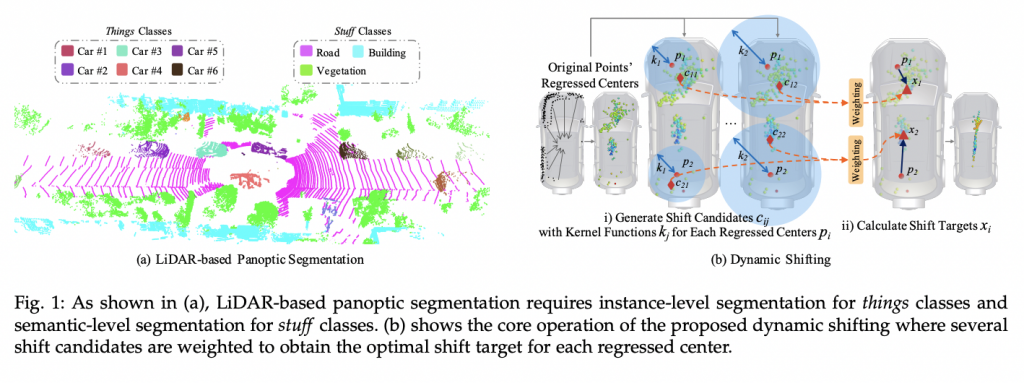
Researchers have now extended the single frame version of DS-Net to the new challenge of 4D panoptic LiDAR segmentation. Not only does the task necessitate panoptic segmentation for each frame, but it also necessitates consistent objects IDs across frames. To do this, the team offers temporally unified instance clustering on aligned and overlapped subsequent frames to achieve simultaneous instance segmentation and association. The final 4D panoptic LiDAR segmentation findings are formed by fusing temporally consistent instance segmentation with semantic segmentation.
SemanticKITTI and nuScenes are two large-scale datasets on which the researchers conduct experiments. On SemanticKITTI, the team also investigates the extension of 4D panoptic segmentation. SemanticKITTI is the first dataset to describe and benchmark the challenge of LiDAR-based panoptic segmentation. SemanticKITTI has a total of 23,201 training frames and 20,351 testing frames. The team might build instance labels by assigning instance IDs to points inside bounding boxes using the point-level semantic labels from the recently released nuScenes lidarseg challenge and the bounding boxes provided by the detection job.
In both validation and test splits, DS-Net surpasses most existing approaches by a substantial amount. In most criteria, DS-Net exceeds the best baseline technique. In PQ and PQTh, DS-NET outperforms the best baseline approach by 2.4 percent and 3.5 percent, respectively. nuScenes, unlike SemanticKITTI, has incredibly sparse point clouds in single frames, which makes panoptic segmentation even more challenging. The findings support the DS-Net’s generalizability and usefulness.
CONCLUSION
The team is one of the first to tackle the task of LiDAR-based panoptic segmentation with the goal of delivering a comprehensive vision for autonomous driving. Researchers propose the innovative DS-Net, which is specifically developed for successful panoptic segmentation of LiDAR point clouds, to address the difficulty posed by non-uniform distributions of LiDAR point clouds. The consensus-driven fusion module and the unique dynamic shifting module both benefit from DS-strong Net’s baseline design. Further analysis demonstrates the dynamic shifting module’s robustness as well as the interpretability of the learned bandwidths.
Paper: https://arxiv.org/pdf/2203.07186v1.pdf
Github: https://github.com/hongfz16/DS-Net
Suggested
Credit: Source link
Comments are closed.