Listen to this article ![]() |
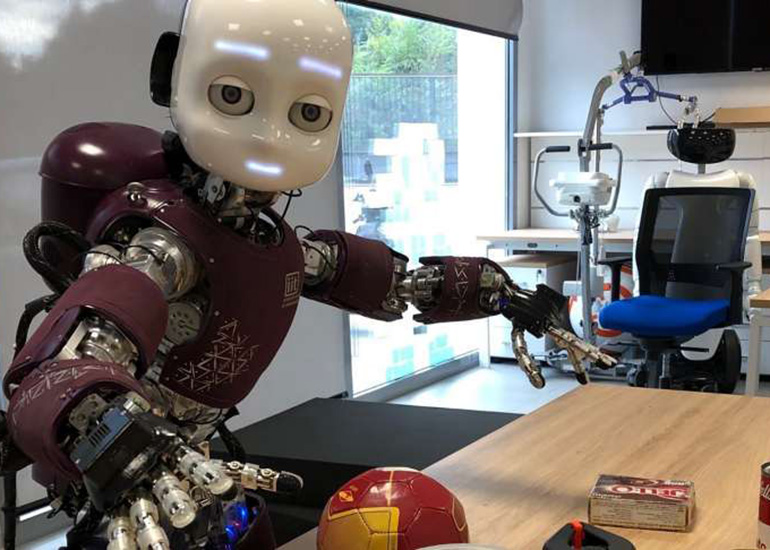
The iCub robot, developed by the Italian Institute of Technology, modeling human learning and development in a real-world setting. | Source: Intel Corporation
Intel Labs, in collaboration with the Italian Institute of Technology and the Technical University of Munich, introduced a new approach to neural network-based object learning that uses interactive online object learning methods. This approach gives robots the ability to learn new objects after deployment autonomously.
The approach is meant to make possible future applications like robotic assistants interacting with unconstrained environments possible. These robots could be at work in logistics, healthcare or elderly care.
The method was tested using a robot in a simulated environment. The robot moves its “eyes”, an event-based camera or dynamic vision sensor, to actively sense objects. When the eyes track objects it creates events that are collected and used to drive a spiking neural network on a Loihi chip.
The spiking neural network architecture on the Loihi chip localizes learning to a single layer of plastic synapses and accounts for seeing objects from different angles by recruiting new neurons on demand. When the robot observes a new object, its SNN representation is learned or updated, and if the object is known, the network recognizes it and gives the respective feedback to the user.
“When a human learns a new object, they take a look, turn it around, ask what it is, and then they’re able to recognize it again in all kinds of settings and conditions instantaneously,” Yulia Sandamirskaya, robotics research lead in Intel’s neuromorphic computing lab and senior author of the paper, said. “Our goal is to apply similar capabilities to future robots that work in interactive settings, enabling them to adapt to the unforeseen and work more naturally alongside humans. Our results with Loihi reinforce the value of neuromorphic computing for the future of robotics.”
Using the newly developed methods, Intel’s team was able to demonstrate continual interactive learning on the company’s research chip, Loihi. The chip used up to 175 times less energy to learn a new object, with similar speed and accuracy, as conventional methods running on a central processing unit (CPU).
The team’s research was published in the paper “Interactive continual learning for robots: a neuromorphic approach.”
Credit: Source link
Comments are closed.