Listen to this article ![]() |
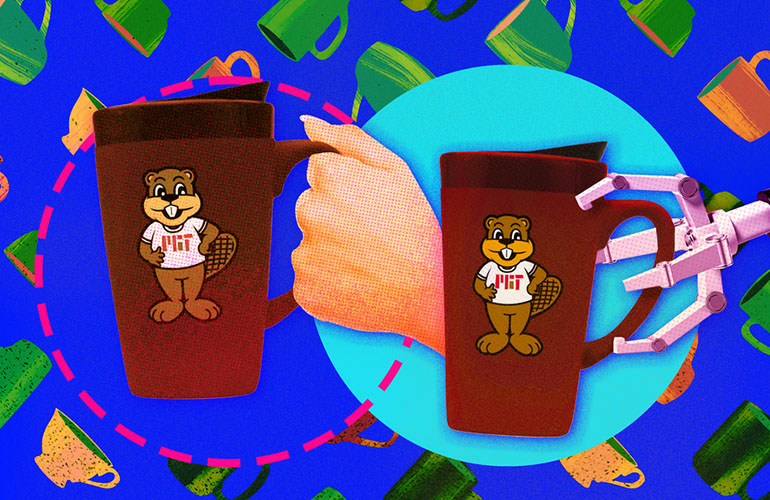
Researchers at MIT have developed a system that allows people without technical knowledge to fine-tune a robot’s ability to perform tasks. | Source: MIT
A group of researchers at MIT have developed a framework that could help robots learn faster in new environments without needing a user to have technical knowledge. This technique helps users without technical knowledge understand why a robot might have failed to perform a task and then allows them to fine-tune the robot with minimal effort.
This software is aimed at home robots that are built and trained in a factory on certain tasks but have never seen the items in the user’s home. While these robots have been trained in controlled environments, they can often fail when presented with objects and spaces they didn’t learn in.
“Right now, the way we train these robots, when they fail, we don’t really know why. So you would just throw up your hands and say, ‘OK, I guess we have to start over.’ A critical component that is missing from this system is enabling the robot to demonstrate why it is failing so the user can give it feedback,” Andi Peng, an electrical engineering and computer science (EECS) graduate student at MIT, said.
Peng collaborated with other researchers at MIT, New York University, and the University of California at Berkeley on the project.
To tackle this problem, the MIT team’s system uses an algorithm to generate counterfactual explanations whenever a robot fails. These counterfactual explanations describe what needed to change for the robot to succeed in its task.
The system then shows these counterfactuals to the user and asks for additional feedback on why the robot failed. It uses this feedback and the counterfactual explanations to generate new data and it can use to fine-tune the robot. This fine-tuning could mean tweaking a machine-learning model that has already been trained to perform one task so that it can perform a second, similar task.
For example, imagine asking a home robot to pick up a mug with a logo on it on a table. The robot might look at the mug and notice the logo and be unable to pick it up. Traditional training methods might fix this kind of issue by having a user retrain the robot by demonstrating how to pick up the mug, but this method isn’t very effective at teaching robots how to pick up any kind of mug.
“I don’t want to have to demonstrate with 30,000 mugs. I want to demonstrate with just one mug. But then I need to teach the robot so it recognizes that it can pick up a mug of any color,” Peng said.
This new framework, however, can take the user demonstration and identify what needs to change about the situation for the robot to work, like possibly changing the color of the mug. These are the counterfactual explanations presented to the user, who can then help the system understand what elements aren’t important to complete the task, like the color of the mug.
The system uses this information to generate new, synthetic data by changing these unimportant visual concepts through a process called data augmentation.
MIT’s team tested this research with different human users, as this framework makes them an important part of the training loop. The team found that users were able to easily identify elements of a scenario that can be changed without affecting the task.
When tested in simulation, this system was able to learn new tasks faster than other techniques and with fewer demonstrations from users.
The research was completed by Peng, the lead author, as well as co-authors Aviv Netanyahu, an EECS graduate student; Mark Ho, an assistant professor at the Stevens Institute of Technology; Tianmin Shu, an MIT postdoc; Andreea Bobu, a graduate student at UC Berkeley; and senior authors Julie Shah, an MIT professor of aeronautics and astronautics and the director of the Interactive Robotics Group in the Computer Science and Artificial Intelligence Laboratory (CSAIL), and Pulkit Agrawal, a professor in CSAIL.
This research is supported, in part, by a National Science Foundation Graduate Research Fellowship, Open Philanthropy, an Apple AI/ML Fellowship, Hyundai Motor Corporation, the MIT-IBM Watson AI Lab, and the National Science Foundation Institute for Artificial Intelligence and Fundamental Interactions.
Credit: Source link
Comments are closed.