Listen to this article ![]() |
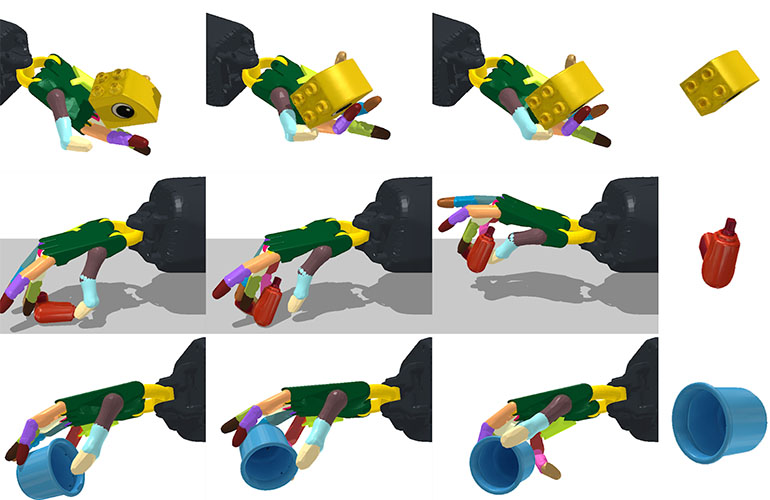
CSAIL’s program teaches robotic hands to manipulate objects facing upwards and downwards.
Most robotic hands don’t look or operate like human hands. They’re designed for limited, specific purposes, and need to have intimate knowledge of the object they’re handling.
Researchers at the MIT Computer Science and Artificial Intelligence Lab (CSAIL) are working to create a framework for a realistic, robotic hand that can reorient over 2,000 different objects without prior knowledge of the object’s shape.
There are two core frameworks at play in CSAIL’s program: student-teacher learning and gravity curriculum.
Student-teacher learning is a training method in which researchers give a teacher network specific information about an object and its environment. The teacher learns information that a robot wouldn’t easily be able to gather in the real world, like the specific velocity of an object.
The teacher then gives this information to the student in the form of observations that a robot could make in the real world, like depth images of an object and joint positions of the robot. The student network then has the ability to learn from those observations and apply those techniques to a number of objects.
It was important to CSAIL’s team that a robot could handle objects with its hand facing upwards or downwards, which required extra training. Robots struggle to handle objects when having to counteract gravity and without the support of a palm under an object.
Scientists taught the robot to counteract gravity gradually. First, they learned in a simulation without gravity. Then, researchers incrementally began to account for gravity, giving the simulation more time to learn how to hold objects even when gravity is at play.
With these frameworks, researchers found that their program was able to learn strategies for holding and manipulating objects that don’t rely on knowing the specific shape of the object.
“We initially thought that visual perception algorithms for inferring shape while the robot manipulates the object was going to be the primary challenge,” said MIT professor Pulkit Agrawal, an author on the paper about the research. “To the contrary, our results show that one can learn robust control strategies that are shape agnostic. This suggests that visual perception may be far less important for manipulation than what we are used to thinking, and simpler perceptual processing strategies might suffice.”
CSAIL isn’t the first research lab to try to create anthropomorphic robot hands that operate like human ones. In 2019, OpenAI developed a program that trained a robot hand to solve a Rubik’s Cube.
While other developers had already trained robots to solve Rubik’s Cubes in seconds, OpenAI looked to train one to solve it without already knowing all possible orientations and combinations first. OpenAI researchers hoped teaching a robot to solve a Rubik’s Cube could help it to develop dexterity that could be used in handling a variety of objects. However, OpenAI recently disbanded its robotics research team due to the lack of large enough data sets to effectively generate reinforcement models.
CSAIL’s program was the most effective with simple, round objects, like marbles, with an almost 100% success rate. Not surprisingly, the program struggled the most with complex objects, like a spoon or scissors. The success rate for objects like these was 30%.
CSAIL’s program operated entirely within simulated scenarios, but the researchers are optimistic the work can be applied to real robotic hands in the future.
CSAIL’s full research can be found here.
Credit: Source link
Comments are closed.