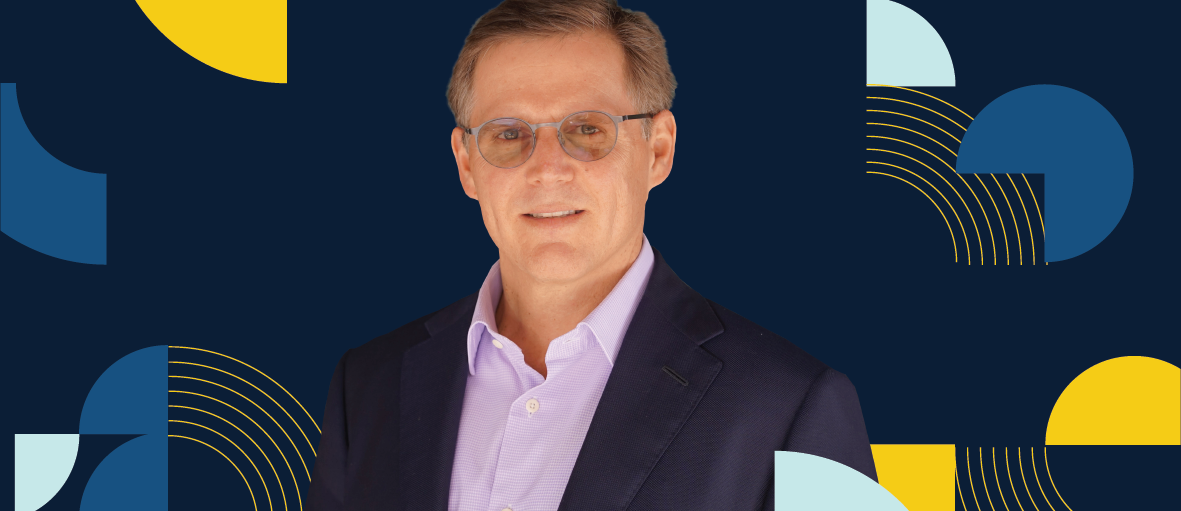
After two decades inside Renaissance Technologies, the legendary quantitative hedge fund founded by James Simons, David Magerman was ready for something different.
His chance came after a public falling out with Robert Mercer, co-CEO of Renaissance at that time, which left Magerman without a job. Fast forward five years, and Magerman co-leads Differential Ventures, a VC firm that invests in enterprise data startups and is raising its second fund with a $60 million target.
In the course of that journey, Magerman has developed a theory on when quantitative methods can work in VC and—as is often the case—when they can’t.
PitchBook recently spoke with Magerman to hear how investors should be thinking about the limits and opportunities of using data-driven strategies in VC.
This interview has been condensed and edited for clarity.
Pitchbook: How does your background inform your view of using data in venture investing?
Magerman: I worked in data-driven approaches to natural language processing back in the 80s and 90s—before it was cool. And I spent 20 years at Renaissance Technologies building systems that tried to extract signals from market data and trying to deploy them.
I’ve seen the overpromise and under-delivery of AI throughout the decades in different scenarios. I’ve also seen how to make it work. Renaissance obviously was very successful at deploying statistical models, but in a much more limited way than people give them credit for. There’s a lot of speculation about how Renaissance does what they do. And the truth is that data science requires consistent data over a long period of time, with enough out-of-sample data to test hypotheses, to generate statistical significance, and also enough discipline that you’re not overfitting your solutions to the data even after you’ve got valid test data.
When it comes down to something like venture investing, there’s really no way to keep generating test data. In statistical arbitrage for stock trading, every day generates an enormous number of new data points, which allows you to test theories. In venture investing, it takes years to generate new data. A company forms with whatever statistics, factors or features you might want to use to predict, and it may take five to 10 years to prove out whether that company is successful or not. And the factors that go into that success are also hard to measure.
With the limitations that you laid out, where is the opportunity for VCs to use data?
I think it’s more of an art than a science. If you can’t trust statistical significance measures and traditional metrics of doing the kind of machine or data science you typically do, it’s more a matter of trying to maximize the value of your data—looking at factor models, trying to extract features that are correlated with success and then deciding for yourself whether you think that those features’ correlation is indicative of causality or just correlation or coincidence.
What data points do you think more venture firms should be watching?
At this point, we don’t do a lot of statistical modeling for our investment strategies because I don’t believe there’s a data set out there that would represent the right training data to even explore whether there’d be correlations, much less another test set that we could use that isn’t overfit.
What we think about is looking at the overall human-generated pattern matching of what we’re seeing in deal flow. We see maybe 5,000 pitch decks a year. That starts becoming enough data that you could think about doing factor modeling on elements of those pitch decks, elements of the backgrounds of the founders. If they’re past the seed stage, you can look at the funds that participated in rounds from PitchBook.
And if we thought it was a valuable exercise, we could then generate models that tell us something about not so much predicting success or failure of a company, but just characterizing the market and then looking at deviations from that. If we see companies typically having founders from certain kinds of colleges, having certain kinds of degrees, teams distributed with a certain diversity of backgrounds, both educationally and otherwise. And then we can see how that company fits into some of those measures.
Again, not to say that that’s predictive of success. Just to say, what’s different about this company compared to other companies?
Pattern matching is often blamed for the lack of diversity in venture or the tendency to back founders from the same cohort of schools. Could that kind of modeling be used to help find companies that maybe don’t fit the mold?
The factor model shouldn’t be assumed to be correlated with success—or failure for that matter. It’s a representation of the data. So if you found that that 70% of the companies came from top-100 ranked universities, and then you found a pitch deck where the founders were not in that category, that would be an indication of a difference.
The question is, is that difference a positive, negative or neutral? And then you could dive into that difference and say, well, this founder has a very unique background, which would allow them to be perhaps more valuable in understanding the problem they’re solving than a traditional path.
You don’t have enough data to actually draw conclusions. But you can then go through anecdotally, and look at the decks that are similar to this one versus the ones that are different. You can decide, from a creative perspective: “Do I think this deck is different in a positive way, or the same in a positive way, or different in a negative way, or the same in a negative way?” That’s a way that you can use data to analyze something where you don’t have a predictive ability, but you can at least use the combination of the preponderance of data, factor modeling and then human intuition.
What data methods are available at the early stage when there’s very little known about companies?
I think the most effective use of data in venture investing is the identification of companies that are either in stealth mode or starting to raise money. There are a lot of companies out there now that are building tools that help you to find investment opportunities.
Do you think that later-stage venture firms should be imitating the approach taken by stock picking entities like hedge funds?
It’s a research question. At Renaissance, we didn’t really know if our quantitative methods would work on stock trading in different markets until we built a model, started trading them and saw what happened.
I think it’s more valid in those later stages because there’s much more detailed, audited, verified data for later stage. It’s still not public data, so you have to trust the sources you’re getting the data from. There are definitely biases in the data because you’re more likely to get data from companies that are successful than ones that fail.
It’s a complicated problem that would require a lot of research. And you’d have to be convinced enough that you’d be successful to spend that many resources on the research to begin with.
Do you think this is the direction private investing is going, that it will become more quantitative over time?
I think that the early stages will not get very quantitative because I think the skill sets that are selected for quantitative modeling don’t have as much overlap with the skill sets required for evaluating early-stage investments.
I’ve learned that myself, seeing how little of my experience at Renaissance has been useful to me in venture investing. There’s just not a lot of overlap.
At the later stages, each investment is worth so much more, and the funds tend to have the capacity to build big teams, that I could imagine you could spend money to hire a team to explore whether you could actually make money doing it that way.
I think the one thing that set Renaissance apart from the beginning is how much the leadership team—including Jim Simons, Peter Brown and Bob Mercer—trusted the math. There were so many times where when things went a little bit crooked, and things stopped working, where if you didn’t trust the math, you would start sticking your finger in and you’re using human judgment or changing the models even though the statistics didn’t justify it.
You’d have to have a similar level of conviction at whatever stage you want to apply statistical models to, to see it through. Because there are so many ways you can misinterpret results, both on the positive side and the negative side. If you start seeing positive results, it doesn’t mean you’re right. There’s a lot of luck and timing that goes into any kind of modeling effort. And again, you need hundreds of years of data to really prove out these models.
But given the amount of money that’s deployed at the later stage, and how valuable the investments are, that’s an area where I think the bang for your buck of building a team and trying to do the research may be worth it.
You deployed quantitative methods at one of the most successful quantitative hedge funds ever. Why did you get into venture? Why thrust yourself in this world where data doesn’t have the same power?
I mean, I did get thrown out of the company [laughs].
But I never really wanted to be a quant. I got into it early in my career after not getting the academics job I wanted.
I enjoyed the challenge of solving a hard problem that was very competitive. But it wasn’t very fulfilling. Having gotten to the end of that career after 20 years, I hadn’t really done anything except made money. As a scientist, you want to publish your results. Obviously, I couldn’t publish my results. I’d learned a lot that I couldn’t share. No one could stand on my shoulders. I couldn’t even point to a legacy of success. No one ever leaves Renaissance, so I didn’t have a lot of people, like Tiger Cubs, out there having learned from me doing other things.
When I thought about how I wanted to impact any kind of community after leaving Renaissance, I thought the best way to make up for lost time was to take the things that I learned in my decades of being an engineer, being a software developer, being a data scientist, and try to impact founders who are building new companies and see if that experience would be useful to them.
So far I’ve been fulfilled by that.
Credit: Source link
Comments are closed.