Researchers At Trinity College Dublin and University Of Bath Introduce A Deep Neural Network-Based Model To Improve The Quality Of Animations Containing Quadruped Animals

It’s difficult to make realistic quadruped animations. Producing realistic animations with key-framing and other techniques takes a long time and demand a lot of artistic skill. Motion capture methods, on the other hand, have their own set of obstacles (bringing the animal into the studio, attaching motion capture markers, and getting the animal to perform the intended performance), and the final animation will almost certainly require cleanup. It would be beneficial if an animator could supply a rough animation and then be given a high-quality realistic one in exchange.
Researchers at the University of Bath and Trinity College Dublin have developed a deep neural network-based technique that could help improve animations containing quadruped animals such as dogs. The team found that given an initial animation that may lack subtle details of true quadruped motion and/or contains small errors, a neural network can learn how to add these subtleties and correct errors to produce an enhanced animation while preserving the semantics and context of the original animation.
The team constructed canine animations that span a range of motions but lack the subtle features of actual canine motion and contain errors using a basic IK solver. This IK solver takes constraints from a large canine motion capture dataset for only a small subset of skeleton joints. A feed-forward neural network was trained on a per-pose basis, using these IK-generated animations and the motion capture dataset as the ground truth, to learn the difference between the IK-generated animations and the corresponding ground truth motions.
Typically, animation production goes through several stages: preliminary blocking of the animation, refining pass, and final polish, including clean-up. This approach could assist an animator in moving from the blocking to the refining stages automatically, speeding up the animation process while allowing the animator to recover control for the final delicacy. Jittering in the output animations could result as a result of this as this enhancing method is designed to work on a per-pose basis. Any such jitter, on the other hand, might be reduced using a high-frequency noise filter. It enables the employment of such a system in real-time applications, such as this technology possible.

This approach could potentially be employed in other applications; for instance, because it can be used in real-time, it could be used as part of a quadruped embodiment system. This technology could also be used as part of a virtual reality quadruped embodiment system for humans by extracting constraints for quadruped IK solver from a human actor attempting to behave as a quadruped.
Graphics, animation, machine learning, and avatar embodiment in virtual reality can all be integrated to create a system for the virtual reality embodiment of quadrupeds, allowing gamers or actors to take on the role of a dog. This technology is the first step in achieving that goal.
Paper: https://dl.acm.org/doi/pdf/10.1145/3487983.3488293
Reference: https://techxplore.com/news/2021-11-deep-method-automatically-dog-animations.html
Suggested
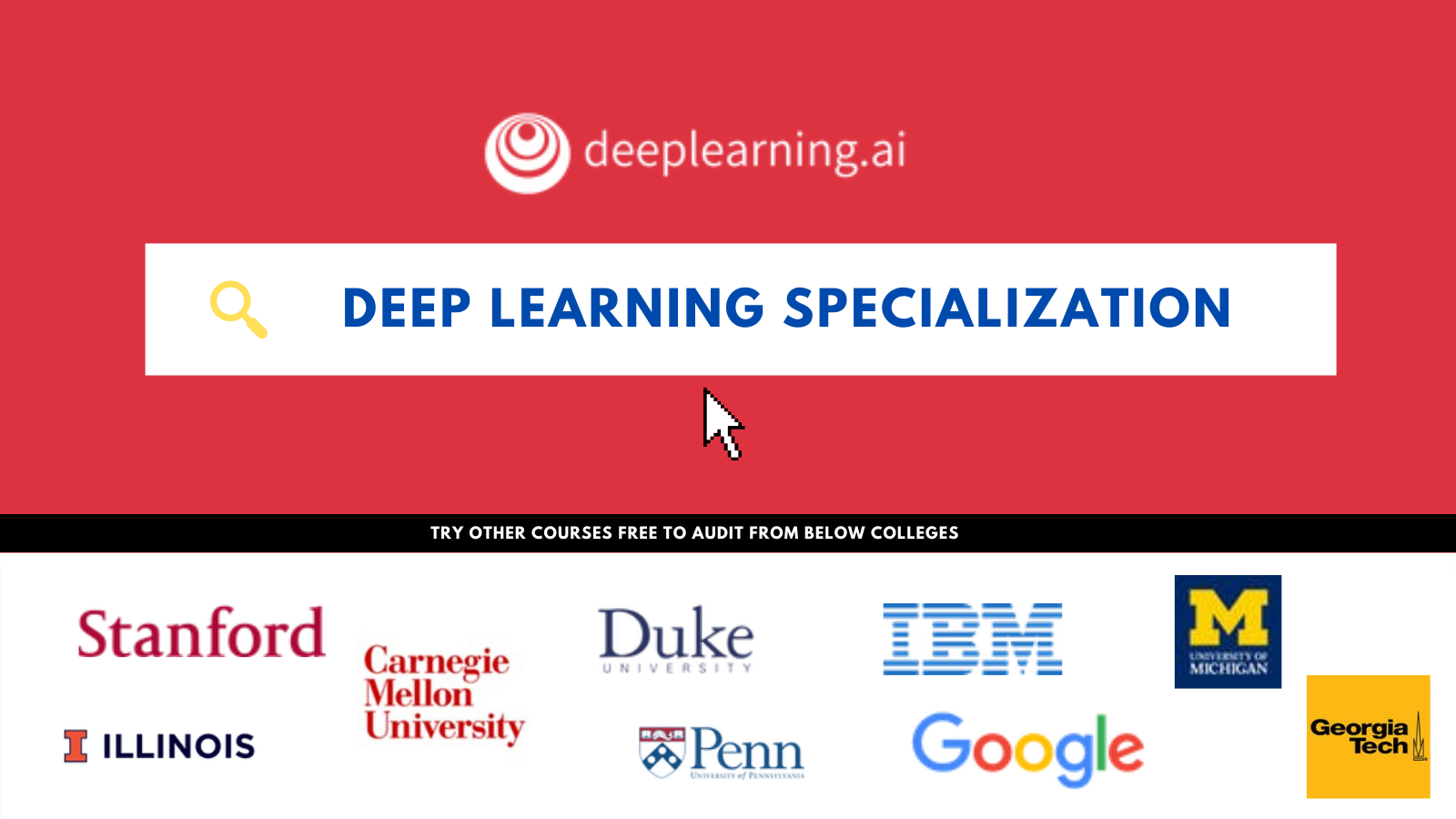
Credit: Source link
Comments are closed.