Researchers From Imperial College London have Developed ‘NeatNet’: A Machine Learning Tool For Robots To Tidy Up Home Environments Similar To An Individuals preference

As robots become more advanced and less expensive, more people may incorporate them into their homes. As a result, several roboticists have been working to create systems that may effectively assist humans with household activities such as cleaning, cooking, and tidying up. Researchers at Imperial College London’s Robotic Studying Lab have just created NeatNet, a novel Variational Autoencoder architecture using Graph Neural Network layers, that will allow robots to clean up home surroundings in ways tailored to individual customers’ tastes. This model is based on a new variational autoencoder structure with graph neural community layers, as described in the pre-published work on arXiv.
“Everyone arranges their house in a unique and particular fashion, which is determined by whether someone is left or right-handed, their aesthetic style, their routines, and even their cultural background,” according to one of the researchers. “We devised a mechanism for learning people’s preferences for how they want their homes to be set up so that a robot might clean it in a customized manner.”
Dr. Johns and his research team created NeatNet, which allows robots to study a customer’s unique cleaning preferences by seeing how they arrange their furniture and belongings in their home. The robots can then utilize these choices as guidance to clean the customer’s houses in ways that reflect their tastes.
NeatNet is inspired by recommender systems, machine learning tools used by streaming platforms (e.g., Netflix, YouTube, Spotify), or other websites to recommend new content to clients. Recommender systems function by analyzing customers’ preferences based on what content they previously viewed, listened to, or accessed.
NeatNet may also analyze the links between different items using a neural graph community. It may, for example, look at how often a keyboard and mouse are placed next to one other or how cutlery is placed on the edge of plates. NeatNet looks upon individual customer preferences in addition to typical object-to-object relationships. It may, for example, look into where the side of the plate consumers usually lay their cutlery, as left and right-handed people may have distinct preferences.
The researchers tested their method in a sequence of trials that used room association examples developed with a tidying simulator to capture the preferences of 75 distinct clients. NeatNet consistently generated tidy and personalized room preparations during these tests. “We discovered that cleaning sceneries in a tailored approach were more satisfying to consumers than cleaning scenes in the same way for everyone,” the Researcher said. “This was true even for basic scenarios, and there are far more alternatives for how to organize each room in real-world homes with hundreds of pieces.”
When robots become more common, their ability to complete tasks in ways that are matched with the preferences of individual person consumers might be pretty valuable. NeatNet may thus prove to be quite beneficial, particularly in increasing the efficiency of home aides and robotics.
NeatNet has been tested in simulations so far, but it has shown to be incredibly promising. The researchers are now working on a follow-up study to put their methods to the test on actual robots and assess it.
Paper: https://arxiv.org/pdf/2111.03112.pdf
Reference: https://techxplore.com/news/2021-11-neatnet-people-tidying.html
Suggested
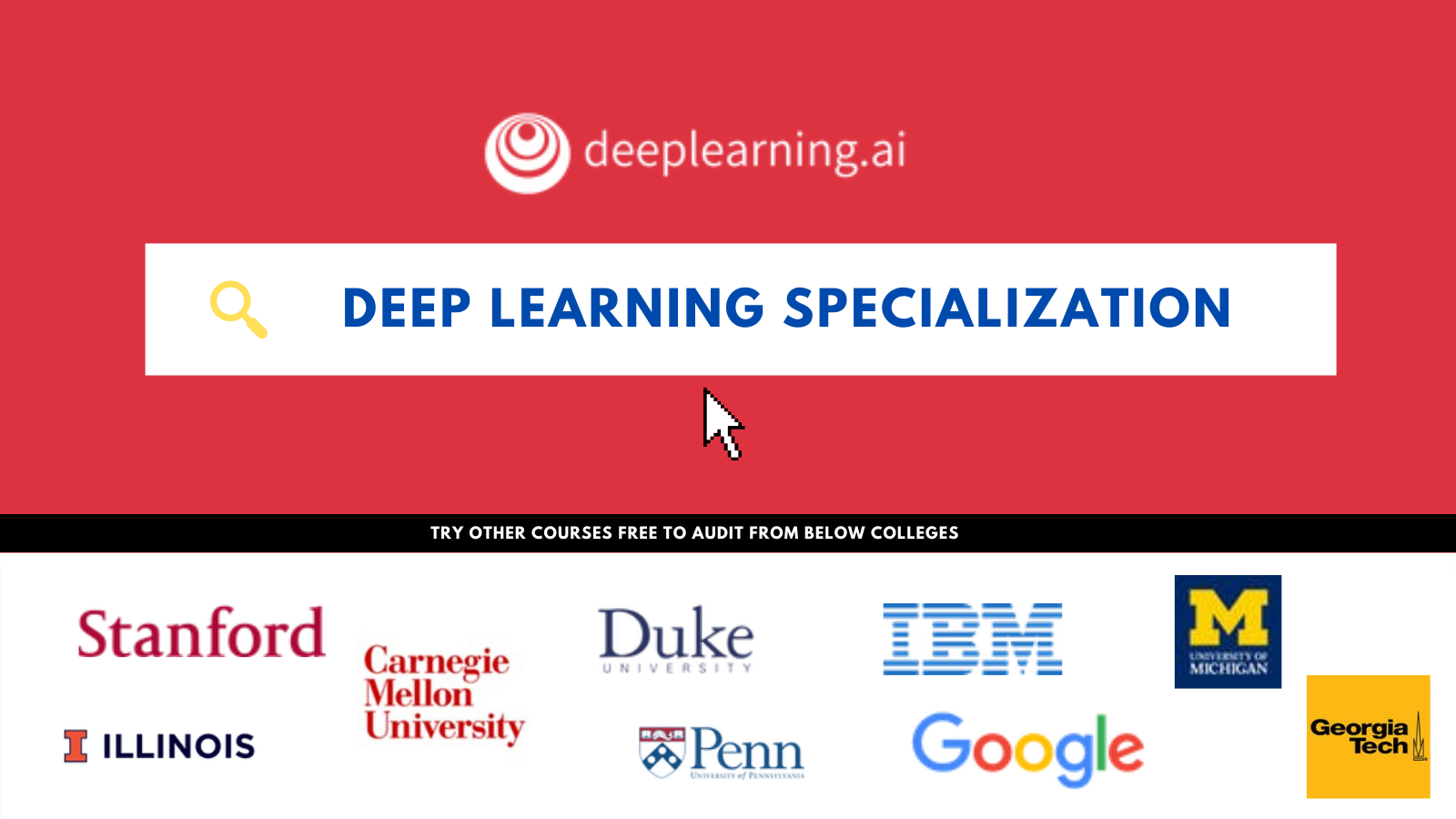
Credit: Source link
Comments are closed.