Researchers From The Hartree Centre, IBM, And REPROCELL Propose An Explainable Machine Learning Approach That Combines Bioinformatics And Domain Insight To Inform Precision Medicine Strategies For Inflammatory Bowel Disease

A project supported by the STFC Hartree Centre Discovery Accelerator accurately predicts patient response to treatments for ulcerative colitis and Crohn’s disease.
Artificial intelligence may soon assist more than 6 million1 individuals worldwide who suffer from inflammatory bowel disease (IBD) in selecting the optimum medication for their illness. An explainable AI pharmacogenomics methodology we created effectively predicted how patients will respond — favorably or negatively — to an IBD treatment 95% of the time, according to research published in PLOSone.
Chronic inflammatory bowel diseases (IBDs) such as ulcerative colitis and Crohn’s disease are caused by clinical, genetic, and environmental variables such as nutrition and lifestyle. Even though all patients have the same symptoms, there is no one-size-fits-all treatment for IBD that is helpful for everybody. Choosing the optimum therapy for a patient is still a trial-and-error procedure for both the doctor and the patient.
According to researchers at IBM Research in the UK and REPROCELL, a stem cell and fresh tissue research firm, used IBD patient data and explainable AI approaches to study treatment reactions with the help of the STFC Hartree Centre’s Discovery Accelerator. Their objective was to discover the optimum medications for IBD therapies less of a guessing game. The resulting collection of algorithms demonstrated that it was feasible to crack the IBD data black box and comprehend forecast and explain how persons with IBD could react to different medications on the market and under development.
Creating a transparent AI pharmacogenomics process
Researchers required IBD patient and medication data for their AI algorithms to generate reasons for their forecasts. TNF release data from IBD patients’ fresh tissue samples, obtained during preclinical treatment candidate testing, was given by REPROCELL. TNF measures reflect inflammation levels; the more significant the TNF level, the more inflammation — and the poorer the medication reaction.
To “learn” from favorable reactions, our algorithm examined data from patients with reduced TNF levels in the presence or absence of medication. It then put the multi-omic, demographic, and pharmacological data from the IBD patients into an “explainable AI pharmacogenomics workflow,” indicating which factors were most important in predicting the efficacy of various IBD medications.
Overcoming the problem of overfitting
Researchers employed two techniques for feature selection before model training to address their data’s “high dimensionality” issue of tens of thousands of genetic characteristics defining a small population of 25 patients.
Firstly, using statistical association analyses, they decreased the dimensionality of their dataset from 33,590 characteristics to around 40 genetic, demographic, and pharmaceutical variables.
Secondly, they selected genetic variants related to Crohn’s disease and ulcerative colitis as input characteristics using biological domain expertise and literature. Overfitting was avoided thanks to this and the use of typical cross-validation approaches.
Their findings revealed differences in medication efficacy based on TNF levels amongst individuals with different demographic, pharmacologic, and genetic characteristics. For example, after treating patients with the anti-inflammatory medication BIRB 796, commonly known as Doramapimod, we discovered novel genetic variants associated with patient responses.
With an error rate of only 4.98 percent on unknown patients, their algorithm predicted the proper medication reaction – for better or worse. These encouraging findings push REPROCELL closer to their aim of reducing the 72 percent of unnecessary adverse medication responses, which might help save costs and lessen patient risk.
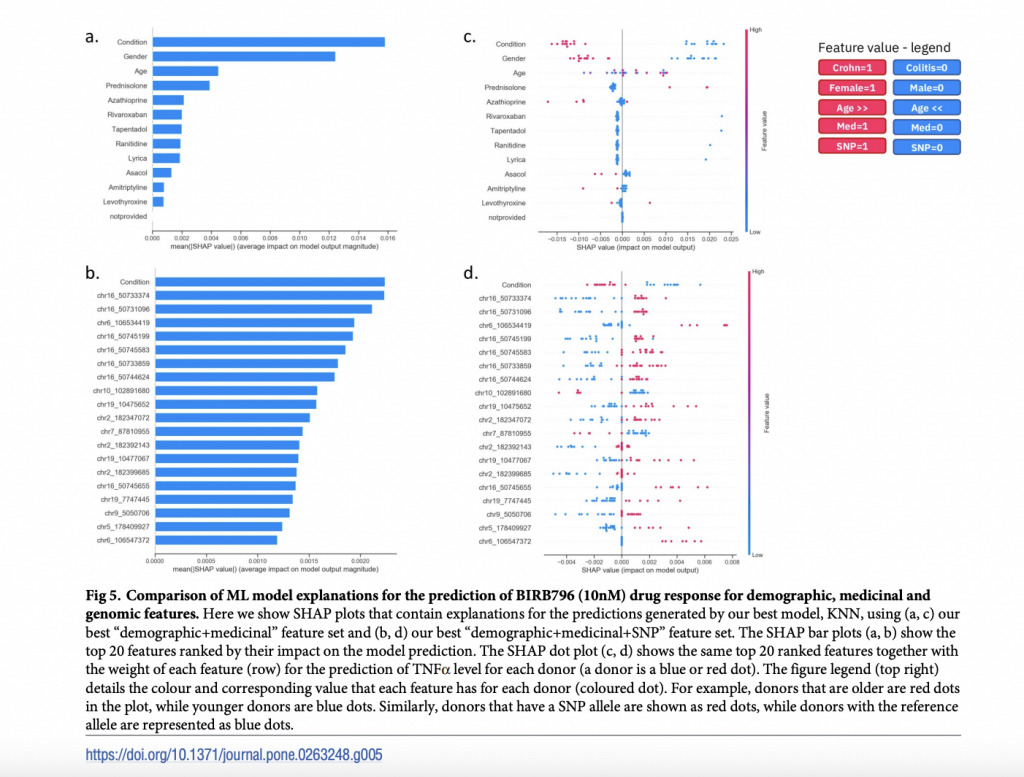
Researchers, for example, discovered which combination of genetic, physiological, or demographic variables can cause someone to react in a specific manner. They utilized their explainable AI to accurately forecast patient medication response based on current REPROCELL data and explain why a patient could respond better or worse to particular therapies. Researchers believe that these prognostic characteristics will be turned into biomarkers in the future to assist screen patients and ensure that they receive the appropriate treatment for their IBD from the start of their treatment.
Their research demonstrates how the explainable AI approach may be used to anticipate various targets and test different medications or mechanisms. The investigation will be extended to a broader group of patients in the future to explore the broader use of our technique and demonstrate its impact.
Paper: https://journals.plos.org/plosone/article?id=10.1371/journal.pone.0263248
References:
- https://research.ibm.com/blog/ai-explains-ibd-drug-treatment
- https://www.frontiersin.org/articles/10.3389/fbioe.2021.635764/full
Suggested
Credit: Source link
Comments are closed.