
Synthetic Aperture Radar (SAR) is an imaging technique that uses a resolution-limited radar system to produce fine-resolution 2D or 3D images. It’s frequently used to gather photos of landscapes or object reconstructions that can be utilized to produce millimeter-to-centimeter scale models of Earth’s or other planets’ surfaces.
When a highly coherent light beam is diffusely reflected on a rough surface, it produces the speckle pattern, a random granular effect. This causes significant changes in the images obtained using SAR techniques, which can impair the quality and interpretability of the images.
Many studies are investigating the use of deep neural network-based algorithms to increase the quality and reliability of SAR data by lessening the speckle effect. While some of these techniques have shown promising outcomes, their effectiveness is still lacking. This is because most existing algorithms learn to de-speckle images through supervised learning. In simple words, they require speckle-free images during training. This can make training difficult, as speckle-free SAR images are often unavailable and must be created or replaced with alternative images.
Researchers from the Polytechnic Institute of Paris and the University of Lyon have recently introduced a new self-supervised learning technique called MERLIN (coMplex Self-supeRvised despeckLINg) for training deep neural networks to decrease speckle effects in SAR data. MERLIN separates the genuine from the ‘imaginary’ portions of complex SAR imagery.
According to the team, the proposed method can be used in any deep neural network architecture. Unlike previously proposed approaches, it is entirely unsupervised and allows researchers to train de-speckling models using single-look complex (SLC) images. Individual picture pixels in SLC images contain amplitude and phase-related information, and they are derived from raw SAR data.
It paves the way for painless and large-scale de-speckling network training by using only a single image and potentially utilizing enormous archives.
MERLIN-trained networks account for spatial correlations caused by the SAR transfer function particular to a certain sensor and imaging mode. Unlike existing methods, MERLIN does not require any extra hypotheses, such as the absence of spatial correlations in the speckle or temporal stability throughout a time series.
In a series of tests, the researchers remark that their training technique could be used to train all types of deep de-speckling networks. Furthermore, even when not trained on speckle-free images, models trained using MELIN produced highly promising outcomes.
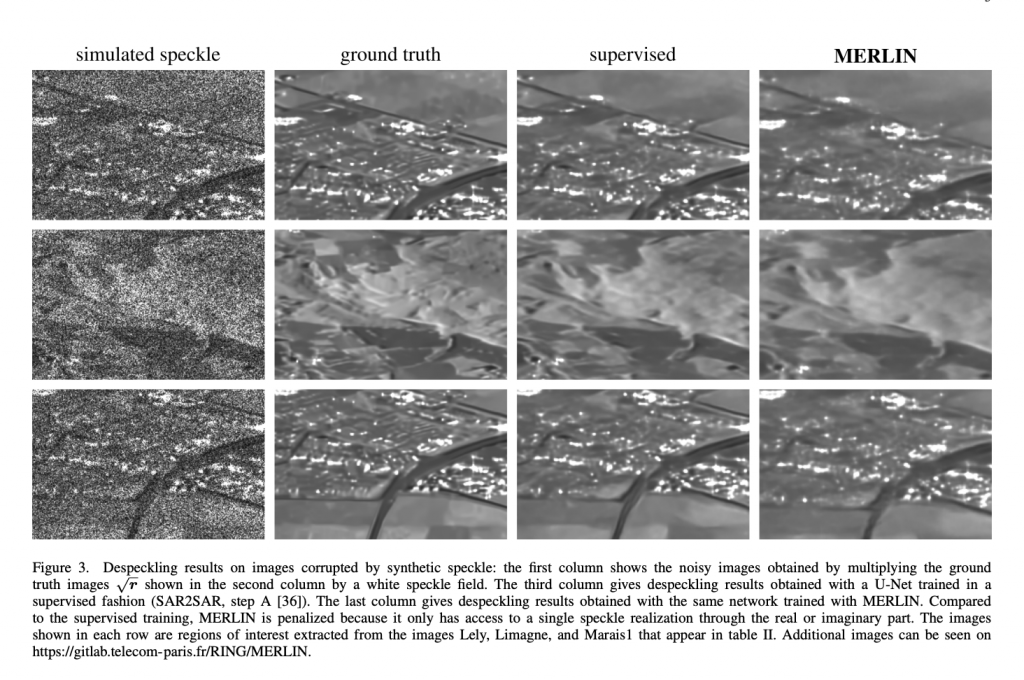
The proposed technique offers an easier and faster way for researchers to train de-speckling models, allowing them to improve the quality of SAR data without having to gather enormous datasets of speckle-free images. It could be extremely useful in geology and other Earth-related disciplines of study in the future.
Paper: https://arxiv.org/pdf/2110.13148.pdf
GitHub: https://gitlab.telecom-paris.fr/ring/MERLIN
Reference: https://techxplore.com/news/2021-11-merlin-self-supervised-strategy-deep-despeckling.html
Suggested
Credit: Source link
Comments are closed.